
Choose Topic

AI Use Cases
A collection of over 250 uses for artificial intelligence
A continually updated list exploring how different types of AI are used across various industries and AI disciplines,including generative AI use cases, banking AI use cases, AI use cases in healthcare, AI use cases in government, AI use cases in insurance, and more

Sign up
to receive a PDF containing all the use cases and stay updated with the latest AI trends and news (you can always unsubscribe)
Blood panel analysis
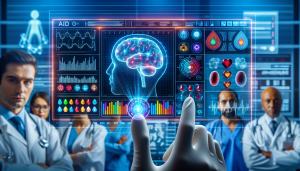
Introduction
There is a rising use of ML and AI in the healthcare sector and these technologies are being used in the various aspects of patient care and medical research. There are, however, other areas that have reportedly benefited from the use of ML and AI and one of them is the analysis of blood panels. Blood panel analysis is one of the most important diagnostic procedures in the contemporary medicine, which helps to assess the patient’s health, identify diseases in the early stage, and control the diseases’ progression. Conventional approach to blood panel analysis requires the involvement of healthcare practitioners in the process of data evaluation, a task that is associated with delays, mistakes and restrictions depending on the expertise of the practitioner. On the other hand, AI and ML algorithms have the capacity to change this process and provide faster, more precise as well as more detailed results.
Challenges
There are however some challenges that affect the use of ML and AI in blood panel analysis even though they pose a lot of potential. First, the integration of these technologies is costly and time consuming; this implies that a lot of investment is needed in terms of finance and time. Second, the healthcare industry is regulated and has legal requirements concerning the privacy of patients’ information, which may hinder the integration of AI and ML. Thirdly, the current health data is not well structured and this makes it difficult to easily collect and analyze the data in the right manner. Fourth, this is because there is a need for a large amount of data to train the ML models and such data can be hard to come by especially due to issues of privacy and ethics. Lastly, if the data used for training the algorithm is not diverse enough to capture the whole spectrum of patients then there is a high likelihood of algorithmic bias.
AI Solutions
There have been several recommendations on how various AI solutions can be implemented to address these issues. Some of the artificial intelligence algorithms include deep learning that can be applied on the blood panel data in order to identify diseases and outcomes. Natural Language Processing (NLP) can be applied to derive important information from the unstructured clinical documents which can be combined with the blood panel results. Also, federated learning makes it possible to develop an ML model using decentralized data while ensuring that data is not exposed. Tempus Labs has leveraged artificial intelligence to interpret clinical and molecular insights such as blood work to suggest the best therapy options. In the same manner, Google’s DeepMind Health has developed an AI that can hinge patient deterioration from blood results. IBM Watson has applied cognitive computing in processing patient information including blood tests to suggest treatment plans.
Benefits
There are a number of ways in which ML and AI can be used in blood panel analysis and all of them present a number of advantages. They can improve the efficiency of the diagnostic process and therefore enhance the quality of treatment. They can also help in identifying the hidden characteristics of the blood panel which may help in identifying diseases in their initial stages. In addition, the use of artificial intelligence and machine learning can alleviate the workload of healthcare practitioners and allow them to attend to the needs of the patients with more complexity. The application of the blood panel results in the development of specific treatment plans for a patient also offers the potential of more efficient and satisfactory treatments.
Return on Investment
From the ROI perspective, the integration of AI and ML in blood panel analysis can be very profitable for the healthcare organizations as it will reduce costs. Better diagnosis and treatment plans help in avoiding other tests and treatments that are not required thus saving on resources. In addition, the technologies aforementioned are capable of identifying diseases at early stages thus avoiding the need for expensive therapies at advanced stages. In addition, the enhancement of the patient experience and outcome will help to improve the image of the healthcare organization and may contribute to more patients and income. However, the ROI may differ considerably by application and the healthcare organizations need to assess the costs and benefits of such technologies carefully.