
Choose Topic

AI Use Cases
A collection of over 250 uses for artificial intelligence
A continually updated list exploring how different types of AI are used across various industries and AI disciplines,including generative AI use cases, banking AI use cases, AI use cases in healthcare, AI use cases in government, AI use cases in insurance, and more

Sign up
to receive a PDF containing all the use cases and stay updated with the latest AI trends and news (you can always unsubscribe)
Bottleneck identification
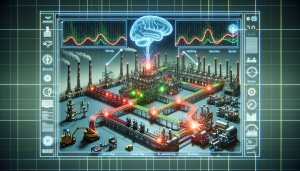
Introduction
In today’s world where time and efficiency are the most valuable assets, the manufacturing sector is always on the lookout for new ways to enhance performance. Another area of interest is the identification of bottlenecks in the production process. Bottlenecks are defined as any constraint in the production line that hinders the overall production and thus affects the efficiency of the whole production process. In recent times, Machine Learning (ML) and Artificial Intelligence (AI) have been used to solve this problem. These technologies have the capability of dealing with large data sets and identifying trends that a human eye may not be able to notice. This has changed the bottleneck detection in the manufacturing sector and has greatly improved the productivity and efficiency of the process.
Challenges
The identification and management of bottlenecks in the manufacturing industry present several challenges. 1) Complex Production Processes: The current manufacturing is characterised by complex processes that include several activities. This makes it difficult to determine where the bottlenecks are. 2) Dynamic Operations: Manufacturing operations are in a state of change and this is because the inputs, processes and outputs vary. This dynamism makes it difficult to determine the bottleneck. 3) Large Volumes of Data: There is a large volume of data generated during the manufacturing process and this data has to be analyzed for identifying the bottleneck. Conventional analysis tools are not very effective in dealing with this type of data and also the analysis done using these tools may not be very accurate. 4) Lack of Real-Time Analysis: In most cases, bottlenecks have to be spotted and dealt with in real time to avoid slowdowns in production. Unfortunately, real-time analysis is difficult as manufacturing operations are complex and ever changing.
AI Solutions
AI and ML offer transformative solutions for identifying and addressing bottlenecks in manufacturing. Predictive analytics leverages vast datasets from different production stages to forecast potential bottlenecks, while real-time analysis allows for their immediate detection and resolution. Additionally, AI algorithms uncover trends that might be overlooked by human analysis, enhancing process optimization and efficiency. By providing actionable insights, AI supports managers in evaluating the outcomes of decisions related to bottleneck management, ultimately streamlining operations and increasing productivity. Beyond addressing bottlenecks, AI and ML enhance several critical aspects of manufacturing. In quality control, AI can analyze production line data to detect defects before products reach customers, reducing waste and ensuring superior quality. Predictive maintenance uses data from machinery to anticipate maintenance needs, minimizing downtime. AI also optimizes production line layouts and operations for uninterrupted material flow. In supply chain management, ML analyzes data to predict disruptions and optimize inventory, ensuring smooth production processes. Together, these advancements significantly improve manufacturing efficiency, reduce costs, and boost overall productivity.
Benefits
There are several advantages of using AI and ML for bottleneck identification in the manufacturing sector. 1) Increased Efficiency: This means that through identifying and controlling bottlenecks, it is possible to enhance the efficiency of production processes. 2) Cost Savings: This in turn results to reduced costs through minimizing on waste and other related expenses. 3) Improved Quality: Through such measures as production process optimization, it is also possible to enhance on quality of the products. 4) Competitive Advantage: This provides a competitive edge to the companies that are using AI for identifying the bottlenecks as they will be more effective and efficient than the competitors.
Return on Investment
The ROI of AI and ML in identifying and solving bottlenecks can be very lucrative. Although, the cost of implementing AI technology can be quite expensive, the benefits that come with it include cost reduction and increased efficiency thereby giving a high ROI. A study by McKinsey revealed that companies that implement AI for process improvement can get a ROI of between 20% and 200%. The ROI will vary depending with such factors as the complexity of the production process, intensity of the bottlenecks, and the quality of the AI solution.