
Choose Topic

AI Use Cases
A collection of over 250 uses for artificial intelligence
A continually updated list exploring how different types of AI are used across various industries and AI disciplines,including generative AI use cases, banking AI use cases, AI use cases in healthcare, AI use cases in government, AI use cases in insurance, and more

Sign up
to receive a PDF containing all the use cases and stay updated with the latest AI trends and news (you can always unsubscribe)
Counting cells in microscope images
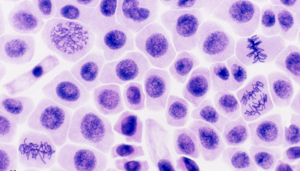
Introduction
Counting cells in microscopic images is a crucial part of current healthcare practice which assists in identification and management of diseases. This has normally been done manually by experts and this makes the process to be error prone and inconsistent since identifying and counting cells is a complicated process. CV and AI have changed this process in the healthcare sector by providing a better way of cell counting which is precise, fast, and reliable.
Challenges
There are however some challenges that are still hindering the growth of CV AI. First, the images of cells are sometimes of low resolution, blurry or noisy due to factors such as lighting, noise or other artifacts, which makes it hard for the AI to provide accurate cell counting. Second, the diversity of cell shapes and sizes as well as the variation in their appearance increases the difficulty of the problem. Third, cells can be superimposed or aggregated, which leads to uncertainty. Finally, to train AI models one needs large datasets which have been manually annotated, a process that is both time-consuming and costly. Some of the real-world challenges include the ‘HPA Cell Atlas project’ through which researchers struggled with the issue of distinguishing the different cell types as well as their spatial relationships.
AI Solutions
These problems are further compounded by the fact that data is often scarce and noisy, and labeling tasks are time-consuming and expensive. In this section, we explore how AI especially in the form of deep learning has the potential to address these challenges. Some of the most effective algorithms include the Convolutional Neural Networks (CNNs) which are capable of learning hierarchical features thus able to cope with the variation in cell appearances. Such strategies as data augmentation and transfer learning can essentially reduce the dependence on large annotated data sets. Some of the specific architecture like U-Nets have been developed to deal with the problem of cell segmentation in complex environments. Advanced techniques such as the one used by Google’s DeepVariant have employed AI to analyse high-throughput microscopy data and have yielded excellent results in the area.
Benefits
There are many advantages of implementing CV AI in cell counting. It also lightens the workload of the healthcare workers and therefore allows them to attend to more challenging issues. This paper also highlights how CV AI is faster, more precise and more reliable than a human counter. In addition, it is able to manage large amounts of data and may reveal information that is not observable by a human investigator. The system can also adapt from previous errors and enhance itself with each new cycle. These advantages are being utilized by organizations such as PathAI with an aim of enhancing pathology.
Return on Investment
The ROI for CV AI in cell counting is quite impressive. It doesn’t just enhance the diagnostic values and speeds up the process but also helps in reducing costs as it decreases the dependence on the manual effort. The predictive analytics that is based on artificial intelligence can also decrease the hospital readmission rates and thereby cutting down the costs. According to a study done by Accenture, AI applications have the potential of creating a value of $150 billion for the US healthcare economy by 2026.