
Choose Topic

AI Use Cases
A collection of over 250 uses for artificial intelligence
A continually updated list exploring how different types of AI are used across various industries and AI disciplines,including generative AI use cases, banking AI use cases, AI use cases in healthcare, AI use cases in government, AI use cases in insurance, and more

Sign up
to receive a PDF containing all the use cases and stay updated with the latest AI trends and news (you can always unsubscribe)
Crop monitoring and yield prediction
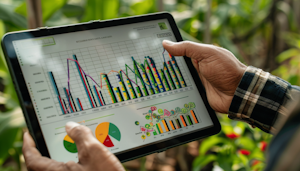
Introduction
One of the oldest and most important occupations of mankind which is also undergoing a change is Agriculture with the help of Artificial Intelligence and Machine Learning. These technologies are used in order to enhance the process of identifying and assessing the condition of crops as well as their potential output, which are both critical to agricultural operations. Starting from the screening of seeds, the observation of the plants’ growth to the forecasting of the yields, artificial intelligence and machine learning are on the verge of changing the approach used by farmers, agricultural scientists, and other players in the agricultural sector. This change is because there is a need to address the issue of inefficiency, low productivity, and the need to feed a growing global population as a result of population increase.
Challenges
There are many challenges that agriculture faces and these are where ML and AI are making a difference. Some of the challenges include variability in weather patterns, pests and diseases, soil erosion, and water issues. Another challenge is the need to produce more food to meet the ever increasing global hunger while at the same time being environmentally friendly. Another problem is the problem of managing large farms and predicting the yields, which is important for planning and decision making for farmers. The traditional ways of monitoring and prediction involve physical assessment and past records which are very cumbersome, laborious and sometimes inaccurate. Also, the absence of real-time information and state-of-the-art analytics tools may result to wrong choices and inefficiencies.
AI Solutions
There are many challenges that are hindering the growth of the agricultural sector in the developing world and Africa in particular. Some of these challenges include climate change, limited access to quality seeds and fertilizers, inadequate financing, and lack of proper infrastructure. AI and ML are offering innovative solutions to these challenges. For example, there is the use of AI-powered drones and satellites to gather information on crop health, soil quality and even weather conditions in real time. The data collected is then analysed using ML models to generate useful information for the farmers. Other applications of AI include the ability of the sector to forecast crop yields with a lot of precision. These predictions are made by using a number of parameters including weather information, type of soil, and the health of the crops. Also, there is the application of AI in the creation of smart irrigation systems that help in the management of water through the use of data. Also, AI has the following applications; AI-powered robots are also being deployed to carry out tasks including weeding, planting and harvesting which helps to cut on costs while increasing production.
Benefits
There are a lot of benefits of using AI and ML in agriculture as well. Firstly, they enhance efficiency through the performance of tasks and the provision of real-time information for decision making. Secondly, they enhance production through the effective utilization of resources and boosting crop yield. Thirdly, they minimize on waste and optimize water usage thus have less impact on the environment. Fourthly, they enhance the ability to engage in proper planning and forecasting since accurate yield estimations are provided. Lastly, they can also assist in enhancing food security by allowing the generation of higher yields at lower inputs.
Return on Investment
It is possible to gain a lot from the implementation of AI and ML in agriculture as well. As per a research report published by Markets and Markets, the AI in agriculture market is predicted to witness a growth of $1 billion in 2020 to $4 billion by 2026 at a Compound Annual Growth Rate (CAGR) of 25.5% during the forecast period. The ROI can be measured in enhanced production, decreased expenses, enhanced performance, and smarter choices. For example, a research done by McKinsey indicated that AI could enhance the yields by 25% through effective water management, optimal use of pesticides and herbicides, and appropriate sowing techniques.