
Choose Topic

AI Use Cases
A collection of over 250 uses for artificial intelligence
A continually updated list exploring how different types of AI are used across various industries and AI disciplines,including generative AI use cases, banking AI use cases, AI use cases in healthcare, AI use cases in government, AI use cases in insurance, and more

Sign up
to receive a PDF containing all the use cases and stay updated with the latest AI trends and news (you can always unsubscribe)
Demand forecasting
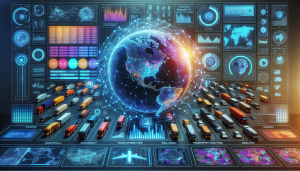
Introduction
Transportation is any one of the industries that are very vast and ever changing. With the new technologies such as Machine Learning (ML) and Artificial Intelligence (AI), there is a change in the transportation and management of transport demand. Demand forecasting is a process of assessing how many passengers or vehicles are likely to be needed at a given time and it plays a very crucial role in the management of transport systems. More and more, these techniques are being applied to improve the quality of these predictions as they employ ML and AI. They analyze the previous data and apply different algorithms to identify trends and provide accurate predictions.
Challenges
There are a number of issues that affect the accuracy of demand forecasting in the transport industry. This is a difficult task because the behaviour of passengers when it comes to travel is rather volatile. Some of the major factors that affect the demand include; weather conditions, time of the day or week, day of the week, season, price, and socio-economic factors among others. Conventional approaches to forecasting do not always have the capability of capturing these nuances. The challenges that are associated with data include quality and quantity. This is because in most cases the data that is used is inaccurate, missing or delayed. Also, handling and organizing this large amount of data can be a big problem. These challenges, together with the fluctuating conditions of the transport industry, make demand forecasting a difficult task.
AI Solutions
There are several challenges that affect the accuracy of passenger demand forecasting in the aviation industry. These include; AI and ML provide good options for these problems. They are capable of working with large data sets and provide predictions that are based on the patterns that are recognized in the data. For example, neural networks which is a type of ML, can be applied in forecasting of passenger demand. They are also capable of identifying complex correlations that exist between different factors that may influence the demand. Also, AI can be applied in data cleaning and preprocessing thus helping to address the problem of data quality and quantity. Also, there is AI solutions such as IBM’s Watson that is capable of doing real time forecasting by updating itself with new data all the time. Also, AI solutions can be deployed in conjunction with other technologies such as the IoT and cloud computing to improve on their efficiency. For example, IoT gadgets can supply real-time information that can be processed by artificial intelligence to give forecasts in real time.
Benefits
There is a wide range of ways in which ML AI can be used in the transport industry for demand forecasting and the benefits are more than obvious. First, it enhances the accuracy of the forecasts that can enable proper resource management and enhanced delivery services. Second, it can improve the efficiency of decision-making since AI is capable of analyzing data and making recommendations much faster than the conventional approaches. This can be important in cases where action has to be taken at short notice, for example, when there are fluctuations in demand. Third, it can help to identify the trends and patterns which may be hard for a human analyst to notice, and thus contribute to better strategic approaches. Finally, it can result in cost reductions since proper demand forecasting can avoid wastage of resources and enhance profitability.
Return on Investment
Investing in AI for demand forecasting can yield significant returns. One study by McKinsey found that AI can reduce forecasting errors by 20-50%, leading to a 65% reduction in lost sales due to product unavailability and a 10-40% decrease in inventory costs. Furthermore, the improved service quality resulting from accurate demand forecasting can increase customer satisfaction, leading to increased revenue in the long run. However, the exact ROI would depend on various factors such as the size and nature of the transport operations, the quality of the data available, and the specific AI technology used.