
Choose Topic

AI Use Cases
A collection of over 250 uses for artificial intelligence
A continually updated list exploring how different types of AI are used across various industries and AI disciplines,including generative AI use cases, banking AI use cases, AI use cases in healthcare, AI use cases in government, AI use cases in insurance, and more

Sign up
to receive a PDF containing all the use cases and stay updated with the latest AI trends and news (you can always unsubscribe)
Diagnosis from patient data
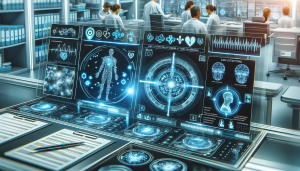
Introduction
It can be seen that the healthcare industry is currently undergoing a number of changes. This transformation is having an effect on the integration of Machine Learning (ML) as well as Artificial Intelligence (AI). These technologies are also applied in the field of healthcare to enhance the quality of the patient care, optimize the clinical practices and minimize the expenses. Another great area of AI use is the diagnosis based on the patient data. By analyzing the large volume of patient data, AI can contribute to the detection of diseases at an early stage, the assessment of individual risks and treatment options, and the enhancement of the overall care process.
Challenges
There are, however, some challenges that can hinder the adoption of AI in healthcare diagnosis even though it has a lot of potential. Some of the challenges include; data privacy and security, data formatting, and the need for massive high-quality data for training AI models. There are also issues of explainability and transparency of the AI algorithms especially when making life threatening decisions in healthcare. Another challenge is how AI will fit into the current healthcare systems as this will need many changes to be made. Also, there is the question of legalization and meeting the set standards since this may hinder the adoption of AI solutions.
AI Solutions
There are various AI solutions which are being designed to solve these problems. For example, there is current research on privacy-preserving machine learning models that can assess patients’ data without infringing on their privacy. Some of the current advances in the AI include designing of algorithms that can operate with varied data and in conjunction with other health care systems. To meet the bar on explainability, there is increasing emphasis on developing AI models that are easy to break down and understand their decisions. With regards to the approval of AI, the developers are collaborating with the regulatory agencies to ensure that the right standards are met. To overcome the problem of the requirement of large data, some techniques like synthetic data generation and transfer learning are used for training the AI models even with limited data.
Benefits
There are a number of ways in which using AI for diagnosis from patient data can be advantageous. Some of the benefits include; AI has the capacity of processing large amounts of information at a time that is faster and with higher precision than a human, this enables the identification of diseases at their early stages. This will contribute to early treatment and enhanced patient results. It also means that individualised treatment plans can be created for each patient based on their data, which in turn should result in better treatments. In addition, AI can also assist in relieving the workload of the healthcare professionals and perform routine tasks so that the professionals can attend to more complicated issues. Finally, AI can also assist in minimizing costs of healthcare through increasing efficiency and minimizing errors.
Return on Investment
It is possible to get a high ROI when AI is applied in diagnosis. A study by Accenture states that AI applications in health can generate a value of up to $150 billion for the US healthcare economy by 2026. The ROI can be generated from different aspects such as cost, revenue, and risk management where cost savings could be realized through enhanced efficiency and reduced errors, revenue could be generated through enhanced patient outcomes and tailored treatments while risks could be minimized through early disease identification and prediction.