
Choose Topic

AI Use Cases
A collection of over 250 uses for artificial intelligence
A continually updated list exploring how different types of AI are used across various industries and AI disciplines,including generative AI use cases, banking AI use cases, AI use cases in healthcare, AI use cases in government, AI use cases in insurance, and more

Sign up
to receive a PDF containing all the use cases and stay updated with the latest AI trends and news (you can always unsubscribe)
Driver behaviour analysis and feedback
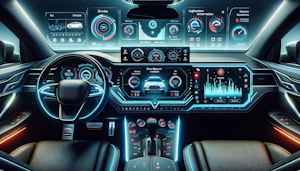
Introduction
The automotive industry is currently undergoing a transformative revolution driven by technologies such as Machine Learning (ML), Natural Language Processing (NLP), and Generative AI. These advancements are not only reshaping the future of mobility but also revolutionizing driver behavior analysis and feedback systems. Traditionally, driver behavior was analyzed using basic parameters like speed, braking, and steering. With the integration of AI, however, the scope of analysis has expanded significantly. AI systems now leverage real-time data from various sensors to provide detailed insights into driving habits, allowing for a more comprehensive assessment of a driver's performance. This real-time analysis helps enhance driver skills, improve road safety, and optimize vehicle performance, signaling a paradigm shift in how driving behavior is monitored and improved. The use of AI in driver behavior analysis opens up numerous possibilities for the automotive sector. AI-driven systems can process vast amounts of data in real time to identify patterns, predict potential risks, and provide actionable feedback. This article delves into the current and future applications of artificial intelligence in driver behavior analysis, highlighting the challenges and opportunities it presents. Furthermore, it explores potential solutions, the return on investment (ROI) for integrating these technologies, and their practical applications in improving safety, efficiency, and overall user experience in the automotive industry.
Challenges
There are however some challenges that the implementation of AI in driver behaviour analysis is likely to face. A major issue is that of data privacy since such systems capture and use information that may be considered private. This data must be protected to ensure that it is not misused in any way. The technology also depends on large, diverse, and high-quality data sets for training the AI models, which may be hard to come by. Also, the reliability of these systems is another issue. Such models rely on correct understanding of the human behaviors, and any wrong perception may lead to giving the wrong feedback. There is also the issue of how to incorporate AI systems with the current automotive systems. This is because it calls for a lot of funding and experience. Finally, there are need for legal frameworks that spell out the rules and who is liable for the use of AI in cars.
AI Solutions
To these challenges, AI offers smart solutions. Such ML algorithms can be trained to identify trends and make predictions about the driver’s behavior and provide the driver with feedback. This is possible because NLP can process human language, thus allowing the communication between the driver and the AI system. With the Gen AI, one can get more adaptive and flexible options that are capable of being changed as time goes on. Some of the modern companies such as Tesla incorporate the use of artificial intelligence and machine learning in real-time processing of data gathered from in-car sensors to monitor the behavior of drivers. Waymo state that they employ AI to perceive the environment and the actions of other drivers, cyclists, and pedestrians. It also can solve the problem of privacy, for instance, by applying the differential privacy and federated learning to preserve the individual data. It can be noted that such companies as Apple apply these techniques to provide the data privacy in their AI systems.
Benefits
There are many advantages that are provided by the AI-driven driver behaviour analysis and feedback systems. They improve safety by determining the riskiness of driving behaviors and warning the driver about them. They also help to create personalized experiences for the user by adjusting to his specific driving habits. These systems can also help in reducing the costs of maintenance as they are able to predict potential problems based on the patterns in the driving. They also support sustainability goals by encouraging the driver to adopt fuel conserving driving behaviors. It also has the potential of enhancing the intelligence of the car to enable other complex features like autopilot and advanced driver assistance systems (ADAS) and the overall experience of driving.
Return on Investment
It is possible to achieve a high ROI when AI is applied in driver behaviour analysis. It thus helps to reduce on costs that are incurred on accidents as well as on the general maintenance of the vehicle through improving the driver’s behavior. For example, a McKinsey report has established that AI has the potential of cutting down the total expenses on vehicle upkeep by 10-20%. It can also generate new revenues with paid features, for example, providing personalized driving tips or advanced driving safety solutions. As for the market analysis, Allied Market Research expects the AI in automotive market to grow from $1.1 billion in 2020 to $12.9 billion by 2027, which proves the potential of the investment.