
Choose Topic

AI Use Cases
A collection of over 250 uses for artificial intelligence
A continually updated list exploring how different types of AI are used across various industries and AI disciplines,including generative AI use cases, banking AI use cases, AI use cases in healthcare, AI use cases in government, AI use cases in insurance, and more

Sign up
to receive a PDF containing all the use cases and stay updated with the latest AI trends and news (you can always unsubscribe)
Drug discovery
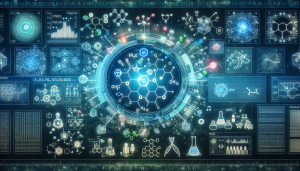
Introduction
It can be said that the pharmaceutical industry has been one of the early adaptors of new technologies in the drug discovery process with the aim of enhancing the efficiency of the process. Other technologies that have been recently adopted include Machine Learning (ML) and Artificial Intelligence (AI). The conventional approach to drug discovery which entails the search for new active compounds is a very slow and costly process. Nevertheless, ML and AI have the possibility of changing this process and offering new ways to deal with big data, to assess the potential of new drug compounds and to enhance drug discovery.
Challenges
There are however some challenges that are still hindering the full realization of the ML and AI potential in drug discovery. One of the major issues is the problem of data; there is no enough quality, diverse and large amount of data for training of ML models. This lack of data can result to generation of wrong biases. In addition, the current state of AI is not able to fully comprehend the dynamics of biological systems and therefore cannot accurately simulate the effect of a drug on such system. It also important to note that there is the issue of how to integrate different type of data (genomic, proteomic, chemical, clinical etc) in a coherent manner that AI can leverage on. Finally, there are ethical and regulatory concerns, including the issues of data privacy and security, and the issue of explaining AI decisions within a regulatory framework.
AI Solutions
There are numerous AI answers to these problems. In silico methods such as deep learning can be employed for the prediction of drug target interactions with the aim of minimizing the costs and efforts of laboratory assays. Also, AI can assist in creating new drug molecules with the desired characteristics through a process called de novo drug design. Furthermore, AI can assist in the identification of adverse effects of drugs so that new drugs can be developed with better safety outcomes. Some of the examples include Atomwise that applies AI for the prediction of bioactivity of small molecules in the drug discovery process and BenevolentAI which applies AI to mine information from the medical literature for potential leads and insights for drug discovery and development.
Benefits
There are a lot of benefits of using ML and AI in drug discovery. It can also greatly minimize the time and the money needed for drug discovery as it can predict the results of experiments even before they are conducted. It also makes it possible to provide tailored treatments as AI can assist in the identification of the right drug for a given patient depending on the patient’s genetics and other characteristics. Also, AI can assist in finding new indications for currently available drugs, which is also known as drug repurposing.
Return on Investment
There are significant return on investment (ROI) for integrating Artificial Intelligence (AI) in drug discovery. A study by the Boston Consulting Group has indicated that AI has the potential of shortening the drug development process and enhancing the probability of discovering new drugs to 10%. This could lead to costs savings of up to $26 billion for each drug. First, there is a significant cost of investing in AI technology and services and the organization has to be very careful when computing the ROI.