
Choose Topic

AI Use Cases
A collection of over 250 uses for artificial intelligence
A continually updated list exploring how different types of AI are used across various industries and AI disciplines,including generative AI use cases, banking AI use cases, AI use cases in healthcare, AI use cases in government, AI use cases in insurance, and more

Sign up
to receive a PDF containing all the use cases and stay updated with the latest AI trends and news (you can always unsubscribe)
Dynamic cloud resource scaling
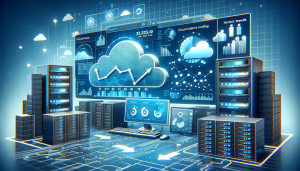
Introduction
The use of cloud resources has been on the rise in the last few years especially in the IT industry. This has made many companies move towards the use of cloud computing since it has helped in increasing business efficiency and productivity. But it can be quite challenging to control these resources in a dynamic environment. This is where it is helpful to use machine learning (ML) and artificial intelligence (AI). ML AI offers smart answers to the problem of dynamic cloud resource scaling and thus enables companies to improve their cloud management and hence increase efficiency and profitability.
Challenges
There are several barriers for dynamic cloud resource scaling. First, it is difficult to forecast when the demand for resources will change. Second, overallocation of resources can cause the waste of resources and high costs, and on the other hand, if there are insufficient resources then performance problems or service interruptions may occur. Third, handling of cloud resources by hand is a labor-intensive and potentially imprecise activity. Fourth, one of the most important issues is how to provide security and comply with all the necessary regulations of the cloud resources. Lastly, there is the problem of how to manage the downscaling of the resources at off-peak periods so that the costs are not incurred unnecessarily.
AI Solutions
These challenges can be addressed by the AI and ML. Predictive analytics can be applied to make accurate demand predictions which in turns helps in proper planning of resources. AI can also assist in the management of resources for example through the application of a ria which reduces the chances of mistakes being made by humans and at the same time freeing up their time to work on other things. In addition, AI can also assist in the security and compliance function by being able to identify unusual events or activities that may not be compliant. There are also types of machine learning models that can assist in the efficient reduction of resources when they are idle. Currently, there is a trend where big names in the cloud such as Amazon Web Services, Google Cloud, and Microsoft Azure are applying the use of AI and ML for dynamic cloud resource management.
Benefits
There are a number of reasons why AI and ML are effective in implementing dynamic cloud resource scaling. Some of the advantages are as follows: cost efficiency due to optimal resource management, enhanced service delivery through demand forecasting, minimization of errors that may occur in the course of work, and improved security and compliance. In addition, the automation of the resource management process enables the IT teams to concentrate on other important activities. Also, the capability to downsize the resources as needed can greatly minimize the costs.
Return on Investment
The ROI of using AI and ML for dynamic cloud resource scaling can be very high. It is a way through which many businesses can realize on savings on resources as well as reducing on wastage of resources. It also helps to increase performance that in turn increases customer satisfaction and may lead to higher revenues. In addition, there are cost benefits that come with automation, security and compliance. Therefore, although a large amount of money may be required to implement AI and ML, the returns in the long run can be profitable.