
Choose Topic

AI Use Cases
A collection of over 250 uses for artificial intelligence
A continually updated list exploring how different types of AI are used across various industries and AI disciplines,including generative AI use cases, banking AI use cases, AI use cases in healthcare, AI use cases in government, AI use cases in insurance, and more

Sign up
to receive a PDF containing all the use cases and stay updated with the latest AI trends and news (you can always unsubscribe)
Dynamic pricing
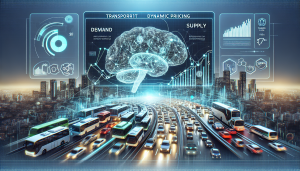
Introduction
The transport industry is one of the most dynamic and evolving sectors globally, constantly demanding innovation and advancements. In recent years, the integration of Artificial Intelligence (AI) and Machine Learning (ML) has revolutionized dynamic pricing strategies, also known as surge or demand pricing. This approach adjusts the prices of products or services based on fluctuating demand. Dynamic pricing is widely applied across various sectors, including transportation, to manage demand and supply, maximize revenue, and enhance customer satisfaction. The transport industry has particularly benefited from this model, as it aligns pricing strategies with real-time market conditions, ensuring both profitability and improved service delivery. Dynamic pricing offers several advantages for the transport industry. It facilitates better demand management by enabling companies to adjust prices based on factors such as time, day, or season. This helps attract customers during peak times while maintaining competitiveness during off-peak periods. The model also enhances revenue by aligning prices with demand patterns, ensuring optimal resource utilization and cost efficiency. Moreover, AI-powered dynamic pricing algorithms provide personalized fare offers, improving customer retention and satisfaction. Real-time price adaptation further ensures that companies remain competitive, responding instantly to changes in demand, traffic, and market conditions. These advancements, coupled with predictive analytics for demand forecasting, enable transport companies to optimize fleet management, reduce operational costs, and balance supply and demand effectively, ultimately creating a more efficient and customer-centric transport network.
Challenges
There are several challenges that the application of ML AI in dynamic pricing within the transport industry has to deal with. The first challenge is the data issue; one of the most important factors that affect the performance of AI and ML models is the quality and the amount of data that is used. The second challenge is the integrativity of the transport industry which is characterized by fluctuating demand, numerous variables that affect the price (location, time of the day, fuel prices), and customers’ behavior. The third challenge is the need for special skills in the implementation and management of the AI and ML models. Also, there is the issue of ethical issues and compliance since many countries have legal requirements on pricing especially when it comes to pricing through AI. This means that if pricing is done using artificial intelligence, it may raise an eyebrow if not done right.
AI Solutions
These challenges have been addressed to a large extent by AI and ML solutions. ML algorithms are capable of dealing with large data sets and thus are able to detect trends and similarities. AI systems can set the prices dynamically depending upon the conditions that prevail at any given time. It is also possible for AI to create different scenarios with pricing and thus help companies come up with the right decisions. Both Uber and Lyft have implemented the use of artificial intelligence and machine learning in their pricing strategies. Uber has implemented machine learning to estimate the demand of riders and thus, change the prices dynamically according to time, location and events. Lyft has also adopted the same technology for their ‘Prime Time’ pricing where the fares are higher when there is high demand.
Benefits
There are a number of ways in which ML AI can be beneficial in the transport industry when it comes to dynamic pricing. Some of the advantages include; enhanced profitability through pricing strategies, enhanced customer satisfaction through equitable and clear pricing and effective demand control. Furthermore, AI can help in cutting down the time and cost involved in reviewing data and resetting prices manually. It also provides more options as prices can be changed in real time depending on the market situation.
Return on Investment
It is possible to realize a high ROI when AI and ML are applied in the area of dynamic pricing. This is because it will help companies to increase their revenues by effective pricing strategies. For example, Uber stated that it enhanced its profit by 9% through dynamic pricing. In addition, by enhancing the levels of customer satisfaction through offering reasonable and understandable prices, companies are able to build a loyal customer base and reduce churn rate which also leads to increased profits in the future.