
Choose Topic

AI Use Cases
A collection of over 250 uses for artificial intelligence
A continually updated list exploring how different types of AI are used across various industries and AI disciplines,including generative AI use cases, banking AI use cases, AI use cases in healthcare, AI use cases in government, AI use cases in insurance, and more

Sign up
to receive a PDF containing all the use cases and stay updated with the latest AI trends and news (you can always unsubscribe)
Employee satisfaction survey analysis
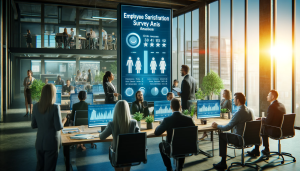
Introduction
Employee satisfaction surveys are an invaluable tool for Human Resources (HR) to understand employees' feelings, attitudes, and motivation levels. These surveys provide rich data that can guide policy decisions and organizational changes. However, the sheer volume of data generated can be overwhelming to analyze manually, limiting the ability to extract meaningful insights. This is where technologies like Machine Learning (ML), Natural Language Processing (NLP), and Artificial Intelligence (AI) prove invaluable. ML, NLP, and AI enable HR departments to process vast amounts of survey data efficiently, identify sentiment trends, and extract critical information. These technologies help uncover actionable insights that might otherwise be missed, providing a clearer picture of employee satisfaction and areas for improvement. By leveraging these tools, organizations can refine their HR policies, foster a more positive workplace environment, and enhance overall organizational effectiveness.
Challenges
The key challenges in processing employee satisfaction surveys include the large amount of data, the difficulty in structuring it and the diversity of data types. Manual analysis is very labor-intensive and also inaccurate. Also, it can be quite hard to understand the responses, especially those that are qualitative, particularly where there are follow-up questions to specific questions. The data can also be contaminated by other factors, respondent bias or the halo effect for instance. There is also the challenge of how to convert these findings into actions, which is a different level of difficulty. Other obstacles are as follows: Other challenges are data privacy and making sure that the responses are anonymous, especially in small companies where the responses of individual employees can be linked to them.
AI Solutions
These challenges can be addressed by AI, ML, and NLP as they provide strong solutions. This is because ML algorithms are capable of handling large data sets and are able to identify trends that could have been overlooked if the data was inspected manually. NLP is able to understand text based responses, determine the affect associated with them, and label them appropriately. The results of these processes can then be combined by AI to give a overall picture. In addition, predictive analytics can predict the future trends on the basis of existing information. For example, IBM’s Watson application is an AI and NLP based solution that analyses unstructured data from the employee surveys in a better manner. The HR team at Google utilizes state of the art tools for data analysis and makes use of AI to analyze survey data in order to make decisions. For instance, Ultimate Software’s Perception is a tool that applies AI and NLP to understand surveys and determine employee satisfaction scores and reasons.
Benefits
AI, ML, and NLP provide significant advantages in analyzing employee satisfaction surveys, enhancing precision and speed while eliminating human error and bias. These technologies allow for in-depth analysis, uncovering patterns that might be missed in traditional methods, and enable predictive analytics to anticipate trends and address them proactively. Furthermore, AI ensures data is securely collected, stored, and processed, offering an added layer of confidence in handling sensitive employee information. By leveraging AI, organizations can refine survey questions, improve relevance, and make data-driven decisions to enhance employee satisfaction, reduce turnover, and boost overall performance. The combined use of AI, ML, and NLP transforms survey analysis by providing insights beyond simple statistics. AI mimics human cognition, ML predicts trends through learned patterns, and NLP processes unstructured text for deeper understanding. Together, these technologies optimize the evaluation of open-ended survey responses and identify individual and collective employee needs. Challenges such as data quality, overfitting, and bias can be addressed through rigorous data cleaning, validation, and employing diverse datasets with regularly updated models. Organizations are encouraged to invest in robust data management and focus on well-defined problems to maximize the potential of AI-powered survey analysis while maintaining fairness, transparency, and reproducibility.
Return on Investment
The return on investment (ROI) for using AI in survey analysis can be significant. While there is an initial investment in AI technology, the long-term benefits often outweigh the costs. Enhanced accuracy and efficiency can lead to improved decision-making and policy development, leading to increased employee satisfaction and productivity. This can, in turn, lead to higher profitability for the organization. Moreover, the predictive capabilities of AI can help prevent costly issues before they arise. A study by IBM found that HR departments that use AI and predictive analytics have 11% higher revenue per employee.