
Choose Topic

AI Use Cases
A collection of over 250 uses for artificial intelligence
A continually updated list exploring how different types of AI are used across various industries and AI disciplines,including generative AI use cases, banking AI use cases, AI use cases in healthcare, AI use cases in government, AI use cases in insurance, and more

Sign up
to receive a PDF containing all the use cases and stay updated with the latest AI trends and news (you can always unsubscribe)
Estimating arrival times
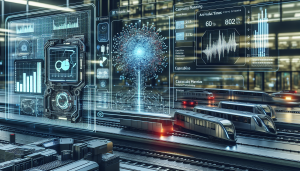
Introduction
The transport industry is vital to the economy, enabling the movement of people, goods, and services. However, predicting arrival times is a persistent challenge due to variables like traffic, vehicle issues, and weather. Machine Learning (ML) and Artificial Intelligence (AI) are transforming the sector, offering data-driven solutions to improve efficiency and accuracy. ML, a branch of AI, uses data to recognize patterns, make predictions, and automate tasks. Applications include route optimization, personalized recommendations, anomaly detection, and predictive maintenance. By integrating ML, the transport sector can enhance operations, reduce costs, and provide better service.
Challenges
The major problem of the transportation industry is the problem of providing the accurate estimated arrival time. Some of the factors that may affect these estimates include; traffic jam, adverse weather conditions, construction related delays, mechanical breakdown of vehicles and human error. Another issue is the large volume of data that is collected from various sources including GPS, traffic flow cameras and sensors. Also, conventional approaches are incapable of working with these large data sets and when applied, they cause delays and errors. Also, there is the challenge of how to incorporate AI and ML into the current systems in the industry, which may be capital intensive and skill intensive.
AI Solutions
AI and ML have played a very vital role in the transportation sector by providing smart solutions to the challenges mentioned above. Such a large amount of data is collected from various sources and such data can be analyzed to make predictions about the arrival time by the machine learning algorithms. For instance, AI can use information from GPS, traffic flow cameras and other devices to determine the traffic flow and provide a better estimate of the time of arrival. The deep learning models help in identifying traffic congestion by considering past trends and the existing situation on the road. Also, it is also possible to estimate when a vehicle will need a breakdown with the help of artificial intelligence by analyzing the data received from the on-board sensors; this way it is possible to perform preventive maintenance and avoid delays. Furthermore, it is also used to plan the best routes in real-time and this may include traffic, weather and road conditions thus providing better arrival time.
Benefits
There are a lot of advantages of using AI and ML in the transport industry. First, it enhances the predictive capabilities of arrival time thus enhancing customer satisfaction and reducing the costs of operation. Second, it facilitates predictive maintenance of the vehicles hence minimizing on unexpected breakdowns and related costs. Third, it adjusts the routes in real time, the least amount of fuel is used and emissions are reduced, thus being friendly to the environment. Fourth, it automates data processing, thus liberating employees to perform more important tasks. Last but not the least, it supports better decision making through providing data-based insights, thus enhancing overall performance and profitability.
Return on Investment
The application of the AI and ML in the transport industry is costly in terms of the technology and personnel. Nevertheless, the benefits are quite impressive. Better arrival time prediction is one of the most effective ways to enhance the customer satisfaction, which in turn yields to customer loyalty and revenue. This paper also found that proactive maintenance can help minimize incidences of unexpected breakdowns as well as the associated costs. Real time route optimization can also help in cutting down on fuel usage and greenhouse gas emissions hence reducing costs and meeting environmental standards. Automated data processing can release employees for more creative activities, thus enhancing their efficiency. Also, it is possible to achieve the following through data-based decision making; greater efficiency and improved profitability. Hence, it can be said that despite the initial costs, there is a good ROI when implementing AI and ML in the transport industry.