
Choose Topic

AI Use Cases
A collection of over 250 uses for artificial intelligence
A continually updated list exploring how different types of AI are used across various industries and AI disciplines,including generative AI use cases, banking AI use cases, AI use cases in healthcare, AI use cases in government, AI use cases in insurance, and more

Sign up
to receive a PDF containing all the use cases and stay updated with the latest AI trends and news (you can always unsubscribe)
Failure prediction
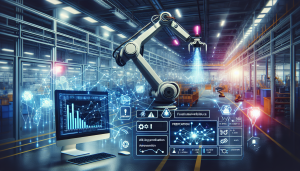
Introduction
The manufacturing industry, a cornerstone of many economies, faces challenges such as equipment failure, production line downtime, and quality defects, all of which can impact operational efficiency and financial performance. The integration of Machine Learning (ML) and Artificial Intelligence (AI) has introduced transformative solutions, enabling manufacturers to detect potential failures before they occur and implement preventive maintenance strategies. These advancements reduce costs, minimize downtime, and improve production quality. Predictive maintenance and AI-driven failure prediction play a vital role by analyzing large datasets to anticipate machinery issues, optimize resource allocation, and ensure continuous operations. This proactive approach has become a game-changer in enhancing efficiency and maintaining competitiveness in the industry. AI and ML have also revolutionized quality control in manufacturing by analyzing data throughout the production process to identify and correct potential defects early. This reduces waste, rework, and customer complaints while boosting operational safety through real-time hazard monitoring. Case studies highlight successful implementations, such as GE's predictive maintenance for aircraft engines, which halved downtime, and Tesla's AI-driven quality control systems, which have significantly reduced manufacturing defects. As technology advances, the future of failure prediction in manufacturing looks promising, particularly with the integration of IoT and real-time data collection. Despite challenges like data privacy concerns, embracing these innovations positions manufacturers at the forefront of industrial transformation, ensuring safer, more efficient, and profitable operations.
Challenges
There are numerous obstacles that are encountered in the manufacturing industry that hinder its effective functioning. Equipment failure is one of the most severe problems that result in unexpected downtime, extended time to complete production, and high costs. This is because it is hard to determine when such failures will occur since the machinery and equipment are quite complex and the operating conditions may also be unpredictable. Other challenges include the problems of ensuring quality of products, managing the supply chain and the shortage of skilled workforce. Also, the vast amounts of data produced by contemporary manufacturing processes present a complex and often unmanageable task for analysis and interpretation.
AI Solutions
There are various ways that AI and ML can be used to tackle these issues. In failure prediction for instance, Machine Learning models can be trained with past information to come up with future failure forecasts. For instance, predictive maintenance models use big data from sensors fitted in machinery in an effort to determine when a specific element is likely to fail. These models search for trends in temperature, vibration among other factors that may be a sign of a fault. In quality control, for instance, AI can be applied in image recognition to detect defects in products during the production process. AI can also be employed in the analysis and enhancement of the supply chain as well as in the recruitment and training of personnel. Some of the well-known AI platforms applied in the manufacturing sector include IBM’s Watson, Microsoft’s Azure, and Google’s Cloud AI.
Benefits
There are numerous advantages of using AI in the manufacturing sector. Some of the most important include; AI based predictive maintenance can cut on downtime as well as the associated costs. It also can help to increase the useful life of equipment by detecting potential problems before they become serious. This paper also found that AI can enhance product quality through quality inspection and defect correction at the early stages of production. With the optimization of supply chain processes, AI can therefore help to minimize on costs as well as increase efficiency. Finally, the use of AI can also assist in solving the challenge of labour shortage by taking over specific functions and in the training of employees.
Return on Investment
AI can provide high ROI in the manufacturing sector. McKinsey in its report stated that AI in predictive maintenance can lower downtime of equipment by between 35-50% while increasing machine life by 20-40%. A research by Capgemini also revealed that AI can enhance productivity by between 15-20% and cut costs by between 15-25%. Still, the exact ROI is not fixed and will vary based on several factors such as the size of the company, the complexity of the manufacturing operations, and the extent of AI adoption.