
Choose Topic

AI Use Cases
A collection of over 250 uses for artificial intelligence
A continually updated list exploring how different types of AI are used across various industries and AI disciplines,including generative AI use cases, banking AI use cases, AI use cases in healthcare, AI use cases in government, AI use cases in insurance, and more

Sign up
to receive a PDF containing all the use cases and stay updated with the latest AI trends and news (you can always unsubscribe)
Financial data synthesis
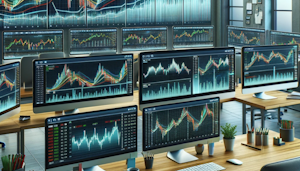
Introduction
The use of Artificial Intelligence (AI) has revolutionized many sectors and financial services are some of the main beneficiaries. One of the latest developments of AI is the Generative AI or Gen AI that is now helping in the creation of financial information. Gen AI is an advanced form of AI that employs machine learning models to create content including text, images, and videos based on the data provided. In the context of the financial services industry, Gen AI can work with large sets of complex financial information to break it down, assess it and even create new data sets for the company, which may change the approach to work of financial institutions.
Challenges
There are however some challenges that are hindering the complete adoption of Gen AI in the financial services industry. These are; the accuracy and credibility of the generated data, data privacy and security, the need for vast amounts of data to train the AI models, the black box issue and the lack of transparency of AI decisions, and the legal and regulatory barriers. For example, JPMorgan Chase’s COIN project which is an AI-powered tool for the legal document review also had issues with data quality and reliability. Also, AI systems such as DeepMind’s AlphaGo need large data sets and processing power which may be difficult for many financial organizations to achieve.
AI Solutions
There are several solutions that can be provided by Gen AI to the problems in financial data synthesis. The quality and the quantity of the synthesized data can be enhanced with the help of AI algorithms that are trained to learn from previous errors. There are AI-enabled data encryption and anonymization methods that can be used to meet data privacy and security demands. This paper also explores how transfer learning and federated learning can minimize the data required for training AI. Explainable AI (XAI) can improve the transparency and accountability of AI decisions. Also, AI can be made such that it follows the set regulations thereby being compliant. For example, the financial services firm, Numerai, applies federated learning in developing machine learning models that analyse the stock market with data being centralised.
Benefits
Gen AI in financial data synthesis can provide the following advantages. It can increase the speed and the precision of the financial data management and processing as well as the decision making. It can also help in handling monotonous tasks, so that the employees can concentrate on challenging activities. With the help of Gen AI, it is possible to offer customized services to customers according to their financial conduct. It can also assist in identification of risks since it can be used in forecasting the financial conditions of the market as well as identifying criminal activities. For example, American Express leverages AI to assess more than one trillion dollars in transactions per year and prevent fraud.
Return on Investment
The ROI of Gen AI in financial data synthesis can be quite impressive. As per Accenture, AI has the potential of increasing profitability within the financial services industry to 31% by 2035. With Gen AI, it is possible to reduce costs and improve the quality of work through automation, minimizing errors and increasing efficiency. It can also increase revenues by improving the customer experience and providing tailored services. The ROI can also be viewed in the context of enhanced risk management and fighting fraud. For example, MasterCard’s Decision Intelligence which is an AI-based fraud detection tool has helped the company to lower the rate of false declines thereby increasing the efficiency of fraud loss prevention and improving the customers’ experience.