
Choose Topic

AI Use Cases
A collection of over 250 uses for artificial intelligence
A continually updated list exploring how different types of AI are used across various industries and AI disciplines,including generative AI use cases, banking AI use cases, AI use cases in healthcare, AI use cases in government, AI use cases in insurance, and more

Sign up
to receive a PDF containing all the use cases and stay updated with the latest AI trends and news (you can always unsubscribe)
Flooding prediction
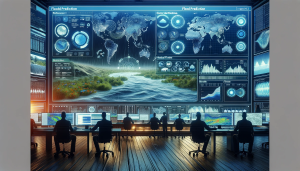
Introduction
Flooding is one of the major natural disasters which occur in different countries and continents on annual basis. As reported by the UN Office for Disaster Risk Reduction, floods impacted 43.4% of the total number of natural disasters occurred between 1998 and 2017, affecting 2.3 billion people and led to $662 billion loss. This is an important challenge for the environmental industry as this is a task that is crucial for the sector. The conventional methods of flood prediction as being based on hydrological models have been seen to be inaccurate and slow. This is where the use of machine learning (ML) and artificial intelligence (AI) have come in as revolutionary concepts. With the help of ML AI, scientists and researchers are working on the development of predictive models that can assist in reducing the impact of flooding through early warning and preventive strategies.
Challenges
It can be seen that predicting floods is not an easy task and there are many issues that can hinder such predictions even with the current technology. First, the problem of data inaccuracy is one of the major factors that affect the accuracy of the predictions. This is because data such as rainfall, river flow, soil moisture and other meteorological and geographical data are not always accurate and of high quality. Second, this is because the hydrological cycle is highly complex and is characterised by several interconnecting factors and non-linearities therefore making it difficult to develop accurate prediction models. Third, there is a lack of real time information which is very important in making flood prediction. Fourth, traditional models do not incorporate the effects of anthropogenic factors including changes in land use and cover on the dynamics of flooding. Finally, there is a need for models that are capable of producing flood predictions that are as precise as possible, and preferably, at a sub-catchment scale, as floods have very varying effects in different areas.
AI Solutions
These challenges are being addressed with the help of AI and ML to improve the flood prediction technologies. AI algorithms which are trained on historical as well as the real-time data are capable of recognizing the trends and forecasting the floods. These models can also take into account numerous factors such as weather conditions, geography, and people’s actions. For instance, Google’s AI-based flood forecasting application integrates machine learning, remote sensing, and digital elevation models and rainfall to determine floods. Likewise, IBM’s The Weather Company applies AI to break down weather information and make weather predictions. Furthermore, AI can assist in the data gathering and processing processes. Drones with AI capabilities can therefore used to gather information on river levels and rainfall in real time while AI algorithms can be used to recognize conditions that may lead to floods.
Benefits
There is a number of advantages of applying AI in flood prediction. Firstly, it increases the efficiency of the predictions which in its turn can contribute to reducing consequences of floods. Secondly, it enables the making of real time predictions therefore facilitating early evacuation and other preventive measures. Third, it is capable of giving specificregional predictions that can assist in decision making and resource management at the sub-regional level. Fourth, AI can assist in the generation and interpretation of data thus improving on the amount and quality of information used in making the predictions. Last but not the least, application of AI can assist in determining the effects of man’s activities on the frequency of floods which in turn will aid in formulation of policies as well as planning of cities.
Return on Investment
It can be costly to set up AI-based flood prediction systems at the initial stage but the rewards are worthwhile. This is mainly because these systems have the capacity to help in avoiding or reducing loss caused by floods. A report by the Global Facility for Disaster Reduction and Recovery revealed that for every $1 invested in disaster risk reduction, there is a return of between $2 and $13. These benefits may include; avoidance of losses, lower disaster response costs and even lives. Furthermore, the application of AI also helps to minimize costs that would have been used for data gathering and processing.