
Choose Topic

AI Use Cases
A collection of over 250 uses for artificial intelligence
A continually updated list exploring how different types of AI are used across various industries and AI disciplines,including generative AI use cases, banking AI use cases, AI use cases in healthcare, AI use cases in government, AI use cases in insurance, and more

Sign up
to receive a PDF containing all the use cases and stay updated with the latest AI trends and news (you can always unsubscribe)
Food ripeness
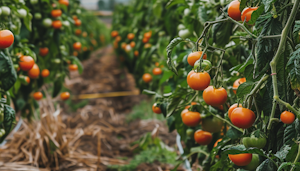
Introduction
Agriculture has been and remains one of the most important sectors of the society as it has provided food and jobs to millions of people throughout the history of humanity. In the recent past, there have been developments which have affected this industry most especially the use of Artificial Intelligence (AI) and Computer Vision (CV). In particular, CV AI has been very relevant in the determination of food ripeness and hence improving on the quality of produce that gets to the consumer. This process entails the use of Artificial Intelligence algorithms to capture images of crops and assess their degree of ripeness. It has revolutionized conventional farming methods by improving the sort and quality of products, decreasing spoilage and enhancing productivity.
Challenges
There are however some challenges that the industry faces despite the promising prospects of CV AI in agriculture. First, the accuracy of the CV AI systems is a function of the quality and quantity of the data that is used for training and this can be a challenge especially in the rural areas. Second, the diversity of the crops and the varying levels of ripeness of the crops make it difficult to train the system, thus needing complex classifiers to distinguish between the different types of crops. Third, the performance of CV AI systems is an unforgiving factor influenced by environmental conditions including lighting and weather. Finally, the expense of integrating CV AI technology, and the absence of skilled personnel, deters the majority of small farmers from embracing such systems.
AI Solutions
There are however several challenges that hinder the effective implementation of food ripeness detection systems including; Despite these challenges, a number of AI solutions have been designed to enhance the accuracy and effectiveness of the food ripeness detection process. For example, CNN based deep learning models have been trained to distinguish between different levels of fruit ripeness with great precision. AgShift and Aclaro Softworks have come up with AI-based solutions that apply Computer Vision to facilitate the quality control process. These solutions not only determine the ripeness of crops but also the quality issues and diseases that may occur in the crops. In addition, some of the startups such as Ripe. io are applying blockchain and CV to establish the concept of the “digital food supply chain” which includes the tracking of the food’s ripeness at every stage of the supply chain.
Benefits
There are several advantages of using CV AI in food ripeness detection. First, it enhances the efficiency of the ripeness detection process thus cutting on the labor costs and increasing the production rates. Second, it enhances the accuracy of the ripeness detection hence only the best quality produce will reach the market. This in turns helps in minimizing on food wastage and hence an increase in the farmer’s profit. Third, it offers important information that can be applied to decide on the right time to harvest crops as well as their transportation. Finally, it supports the concept of sustainable farming as it also helps in the reduction of the use of chemicals in the process of ripening as well as minimizing losses that are incurred in the post-harvest stage.
Return on Investment
It is possible to compute high returns on investment (ROI) when investing in CV AI for the prediction of food ripeness. A research conducted by MarketsandMarkets, state that the AI in agriculture market is projected to be valued at USD 1.0 billion in 2020 and is expected to grow at a CAGR of 25.5% to reach USD 4.0 billion by 2026. This growth is as a result of the rising demand for agricultural products coupled with the requirement for precise crop assessment. There is a possibility of reducing the costs of the farmers, increasing the output of the crops and enhancing the competitiveness of farmers in the market through the application of CV AI systems.