
Choose Topic

AI Use Cases
A collection of over 250 uses for artificial intelligence
A continually updated list exploring how different types of AI are used across various industries and AI disciplines,including generative AI use cases, banking AI use cases, AI use cases in healthcare, AI use cases in government, AI use cases in insurance, and more

Sign up
to receive a PDF containing all the use cases and stay updated with the latest AI trends and news (you can always unsubscribe)
Forecast demand
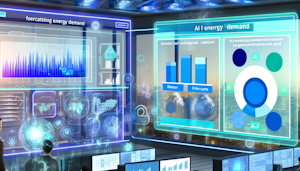
Introduction
The energy industry is a critical driver of global economies, encompassing sectors such as electricity, oil and gas, and renewable energy. It operates in a highly dynamic environment shaped by factors like technological advancements, environmental concerns, and economic trends. A key challenge in this sector is the unpredictability of energy demand, influenced by variables such as population growth, economic activity, and climate change. Traditional demand forecasting methods, which rely on historical data and statistical models, often fall short in capturing emerging trends and complex interdependencies, limiting their effectiveness in predicting future energy needs. Machine Learning (ML) and Artificial Intelligence (AI) have revolutionized demand forecasting in the energy sector by offering greater accuracy, efficiency, and adaptability. These technologies analyze vast datasets to identify patterns, automate forecasting processes, and generate insights that account for seasonal changes, economic fluctuations, and technological advancements. Benefits include optimized resource allocation, integration of renewable energy, and the development of resilient and sustainable energy systems. Central to these advancements is the role of data—historical and real-time metrics—supported by robust integration and management practices. By leveraging ML and AI, the energy industry can address challenges, enhance decision-making, and plan for a sustainable future.
Challenges
There are numerous issues impacting the ability of the energy industry to effectively forecast demand. The first and most important factor is the fact that energy demand depends on many factors that cannot be predicted, such as weather conditions, economic situation or consumers’ consumption trends. Also, the rising use of renewable energy sources which are stochastic in nature, makes the demand forecasting even more challenging. Conventional methods for forecasting fail to capture these complexities and the associated risks and uncertainties efficiently. Also, there are changes in technology, market conditions, and regulation shifts which call for real-time adjustment of forecasting models. Finally, the vast amount of data that is produced by the energy sector can be complex and challenging to deal with using conventional data management tools.
AI Solutions
There are challenges that are faced in the traditional methods of demand forecasting and these include; ML and AI have been identified as possible solutions to these problems. Such characteristics make ML algorithms suitable for dealing with the issues related to energy demand forecasting since they are based on the ability of the algorithms to handle big data, patterns, and relationships. For instance, deep learning algorithms can learn data relationships in a complex manner and therefore are capable of delivering better predictions. Further, the reinforcement learning algorithms are capable of learning from the environment making them very dynamic. Also, AI can help to implement the forecasting process which will minimize time and resources used in coming up with the forecasts. IBM and Google are some of the companies that are already applying AI in demand forecasting. For instance, IBM’s Watson has adopted the use of Artificial Intelligence in forecasting energy demand while Google’s DeepMind has applied the same in predicting wind power generation.
Benefits
There are a number of ways in which AI and ML can be beneficial in energy demand forecasting. Firstly, it can enhance the accuracy of the forecasts that are made, which means that resources are used in a more effective manner and costs are reduced. Secondly, it can deal with large data sets, thus enabling companies to enhance their utilization of data. Thirdly, it is adaptable to changes in the environment, therefore making them more robust than the traditional methods of forecasting. Lastly, it can also help in the automation of the forecasting process hence enabling one to have more time to work on other tasks. For instance, National Grid which is a UK’s electricity and gas utility company stated that it has enhanced the accuracy of its forecast by 20% since it adopted the use of Artificial Intelligence.
Return on Investment
The ROI of applying AI and ML in demand forecasting can be quite impressive. As per a research by the International Energy Agency, a 10 % improvement in the accuracy of the forecast can result in 5 % decrease in the operational costs. This can come to millions of dollars for energy companies that are large in size. Furthermore, enhanced forecast precision can also result in enhanced decision-making, minimized risk, and improved competitiveness. For instance, EON, a European energy company has stated that it has cut down its operational costs by 4% through the use of an AI-based demand forecasting system.