
Choose Topic

AI Use Cases
A collection of over 250 uses for artificial intelligence
A continually updated list exploring how different types of AI are used across various industries and AI disciplines,including generative AI use cases, banking AI use cases, AI use cases in healthcare, AI use cases in government, AI use cases in insurance, and more

Sign up
to receive a PDF containing all the use cases and stay updated with the latest AI trends and news (you can always unsubscribe)
Fraud detection
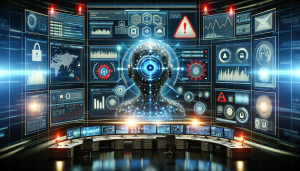
Introduction
In the recent past, the financial services industry has been shaped by the improvement of the technological developments. One of such is Machine Learning (ML), which is a branch of artificial intelligence (AI) that has changed the face of fraud detection. This has been made possible by the increased use of digital transactions which have led to the generation of large data sets that are beneficial as well as hazardous to the financial institutions. Since the conventional ways of identifying fraud are now failing, the use of ML AI in this sector offers a chance for a better solution. The fraud detection systems that are based on ML can process large volume of data in real time, and with the help of learning algorithms, it is able to detect patterns and make predictions about future events. This article aims at explaining the concept of ML AI in fraud detection particularly in the financial services industry, the challenges that are encountered, AI solutions, advantages and real life applications.
Challenges
There are several challenges in fraud detection especially in the financial services industry. The first one is the huge amount of data that has to be processed. This is because the number of transactions that occur within a second is very high and it is therefore very difficult for analysts to keep an eye and identify any suspicious activities. Another challenge is the dynamic nature of fraud. The fraudsters keep on changing their strategies and coming up with new ways of conducting their activities in order to avoid detection, thus making it hard for the organizations to adapt. Another challenge is false positives, where normal transactions are raised as suspicious. This not only causes inconvenience to the customers but also wastes precious time and resources that could have been used to identify real fraud. Finally, the problem of lack of interpretability of the models in ML, makes it difficult to explain why a certain transaction was identified as suspicious, which is important from the regulatory perspective.
AI Solutions
These challenges are also being addressed by AI solutions. Some of the techniques involve supervised learning in which ML models are trained with historical transaction data to learn the difference between normal and fraudulent transactions. The unsupervised learning is also applied to identify the anomalies or patterns in the data that may point to fraud. The ML field, particularly the deep learning branch, is employed to recognize the sophisticated patterns and connections in the data. AI can also minimise false positives through providing a better insight into the context of the transactions. In addition, there is a growing field of explainable artificial intelligence that aims to enhance the transparency of ML models. Finally, the ML can keep on changing to match the ways of the fraudsters by learning from the new data.
Benefits
There are a lot of advantages of applying ML AI in fraud detection. First, it enables real time identification of the fraud which is vital in avoiding monetary setbacks. Second, it minimizes the cases of false positives therefore enhancing the customers’ satisfaction. Third, it is capable of identifying as well as identifying the various and constantly developing schemes of fraud which the conventional techniques are not able to identify. Fourth, it optimises the utilization of resources since the system is able to identify high risk transactions without the need for manual review. Lastly, it is capable of offering a deeper understanding of the nature and dynamics of frauds which may enable financial institutions to enhance their strategies for fraud prevention.
Return on Investment
It is possible to get a high ROI when deploying ML AI for fraud detection. A study by McKinsey & Company revealed that AI can decrease the costs of fraud detection as much as 15%, and increase the efficiency of detection between 30-50%. This is also because the elimination of false positives can also lead to increased customer satisfaction and loyalty. In addition, real-time fraud detection can help in minimizing financial damages. In addition, the analysis of the data provided by AI can enable financial institutions to create more efficient strategies for fighting fraud, which will also decrease expenses in the future.