
Choose Topic

AI Use Cases
A collection of over 250 uses for artificial intelligence
A continually updated list exploring how different types of AI are used across various industries and AI disciplines,including generative AI use cases, banking AI use cases, AI use cases in healthcare, AI use cases in government, AI use cases in insurance, and more

Sign up
to receive a PDF containing all the use cases and stay updated with the latest AI trends and news (you can always unsubscribe)
Homelessness prediction
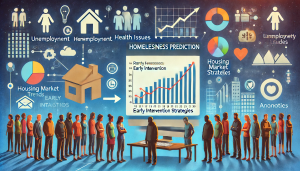
Introduction
Homelessness is one of the most pressing social problems of the modern world that is not limited to Third World countries only but is also observed in developed countries of the West. This is why the forecasting and prevention of homelessness is considered to be an important function of public services. With the recent developments in the field of technology such as machine learning and artificial intelligence, there has been an increasing contribution in this sector. These technologies are utilizing the data-oriented approach to analyze patterns and trends of homelessness so that the public services can plan accordingly.
Challenges
There are several barriers to predicting homelessness and they can be classified into three main groups: barriers related to data, methodological barriers and implementation barriers. Data-related barriers comprise of data gaps, data quality, and data silos as well as data privacy issues. This makes it difficult to identify the root cause of the problem and comes up with proper solutions. Other challenges include; the problem is complicated by methodological barriers such as the inability of current models to capture all aspects of homelessness. Implementation challenges are those which are associated with the need for interdepartmental cooperation, trust in AI, and ethical issues. For example, the city of San Francisco, which has a large tech-savvy population, has failed in the challenging task of predicting homelessness due to the above-mentioned challenges.
AI Solutions
These challenges are addressed by AI and ML that offer predictive analytics, pattern recognition, and decision-making tools. For instance, the Los Angeles Homeless Services Authority (LAHSA) has developed a system known as the Coordinated Entry System (CES) which integrates artificial intelligence and machine learning to identify the needs of homeless people and connect them with the best possible housing solutions. Also, the city of New York has introduced an initiative called Project 8 which applies ML models to identify families who are likely to become homeless and therefore require intervention. In Canada, the Homelessness Partnering Strategy (HPS) has implemented the use of artificial intelligence to determine the order of providing housing to the needy.
Benefits
There are several advantages of using AI and ML in predicting homelessness. They are as follows: It helps in delivering precise predictions to enable focused interventions; it is cost effective in the use of resources; and it is flexible to changes in patterns of homelessness. It also can assist with data issues by being able to analyze large amounts of data and pick out patterns that would otherwise not be seen. In the future, AI can assist in creation of better homelessness prevention strategies and thus to the end of homelessness.
Return on Investment
There is a high return on investment (ROI) when it comes to applying AI in forecasting homelessness. For instance, the adoption of LAHSA’s CES has led to a decrease in the amount of time it takes to provide a homeless person with a home by 45%. This has also been seen in Project 8 in New York, which has also shown great potential for cost efficiency since early intervention on cases of chronic homelessness can be effective in preventing the costs to rise. Although it is often challenging to quantify the ROI in concrete terms because of the nature of the problem and the fact that the benefits can be diverse, such cases prove the concept of high efficiency and cost-effectiveness.