
Choose Topic

AI Use Cases
A collection of over 250 uses for artificial intelligence
A continually updated list exploring how different types of AI are used across various industries and AI disciplines,including generative AI use cases, banking AI use cases, AI use cases in healthcare, AI use cases in government, AI use cases in insurance, and more

Sign up
to receive a PDF containing all the use cases and stay updated with the latest AI trends and news (you can always unsubscribe)
How genes impact health
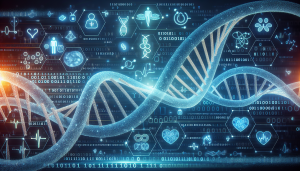
Introduction
Genomics and health are interrelated and this relationship has been the focus of many studies and has resulted to new developments in the medical field. This has led to the increased utilization of genomic data to help explain the genetic basis of diseases, enhance on the development of new therapies and also enhance the quality of health care to patients. Machine Learning Artificial Intelligence (ML AI) has become an important part of the healthcare sector as it assists in the breakdown and evaluation of genomic information. It is capable of working through large amounts of information in a short time, identify relationships that an human may not have been able to make and generate recommendations that can assist physicians in the treatment of patients. Nevertheless, there are certain concerns with the application of ML AI in genomics and health, some of which are concerns to data privacy, data quality and even the correct interpretation of the results from the AI.
Challenges
There are several issues that arise when AI and ML are applied in genomics and health. A major issue is the question of data privacy since genomic data is considered as sensitive information which if misused can have severe implications including discrimination and stigma. As such, the issue of how genomic data is going to be used and patients’ privacy protected is a big issue. Another challenge is data quality. It has been stated that genomic data is high-volume, and it is also susceptible to several factors including genetic variations and environmental factors. It is therefore important to ensure that the genomic data that is captured is accurate and reliable. The other challenge is that, understanding of the outcomes produced by AI is not easy. This is because AI algorithms are capable of creating large datasets and pinpointing various correlations but it is left to the experts to determine the significance of these correlations and how they can be applied to enhance patient care.
AI Solutions
To tackle these challenges, several AI solutions have been proposed. For instance, secure multi-party computation and federated learning are currently employed to prevent data privacy issues when processing genomic data. There are also attempts to enhance the quality of the genomic data through the use of AI algorithms that are able to detect and fix errors, or identify anomalies and variations in the data. Furthermore, AI algorithms are used for analysis of the genomic data where they are able to recognize trends, predict outcomes and suggest findings that may assist the clinicians to make right decisions. These AI solutions aren’t only solving the problems of applying genomic data in healthcare but also opening new horizons in the field of precision medicine.
Benefits
There are several advantages of using ML AI in genomics and health. Firstly, it can enhance the processing of genomic information, which will facilitate the process of developing new cures and enhance the quality of health care services. Second, it can enhance the quality of the genomic data that is collected which means that the diagnoses that are made will be more accurate and the treatments that are given will be more effective. Third, it can offer insights that will assist the clinicians to make better decisions thus enhancing the patients’ outcome and minimizing on the costs incurred in the healthcare sector. Last but not the least, it can facilitate the concept of precision medicine where the treatment is designed according to the genetic disposition of the patient.
Return on Investment
Implementing ML AI in genomics and health can be profitable. A study by McKinsey has stated that AI has the potential of generating up to $100 billion in the healthcare industry on an annual basis by 2025. Such value can be attributed to enhanced patient results, efficient spending of health care resources, and novel income streams from precision therapy. There are cases where the ROI of using AI in genomics and health can be high but this all depends on certain factors such as the quality of the genomic data, the precision of the AI algorithms and lastly, the implementation of the insights derived from AI in the clinical practice.