
Choose Topic

AI Use Cases
A collection of over 250 uses for artificial intelligence
A continually updated list exploring how different types of AI are used across various industries and AI disciplines,including generative AI use cases, banking AI use cases, AI use cases in healthcare, AI use cases in government, AI use cases in insurance, and more

Sign up
to receive a PDF containing all the use cases and stay updated with the latest AI trends and news (you can always unsubscribe)
Livestock prediction
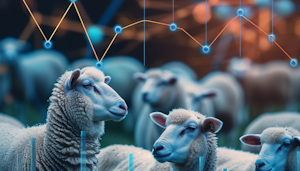
Introduction
Livestock farming has been one of the key pillars of agriculture since the early days of human civilization and has played a crucial role in the development of human societies. Nevertheless, the sector is currently in the middle of a revolution, which is being brought about by the application of Machine Learning (ML) and Artificial Intelligence (AI). These technological developments are thus providing predictive capabilities that enable the enhancement of the efficiency and productivity of livestock management. Some of the areas that have been affected include disease prediction, breed selection, and feeding regimes among others, and ML AI is changing the dynamics of the livestock industry for the better for farmers and other stakeholders in the agriculture sector.
Challenges
It should be noted that using ML AI in the livestock industry has its own set of challenges and issues. Some of the challenges include; poor development of digital infrastructure in the rural areas, low level of AI literacy among the farmers and fears on the data privacy. Other barriers are; the expense of AI technology, challenge in capturing quality data for the AI models, and the variability associated with external conditions that affect the health and output of livestock. In addition, there are regulatory obstacles in the use of AI in agriculture, including the issues to do with data ownership and protection of animals.
AI Solutions
There are different strategies being implemented to address these issues by applying various AI solutions. For example, predictive models that are powered by artificial intelligence are applied in the prediction of diseases which assist the farmers to prepare. There are numerous applications of machine learning in the livestock industry including the analysis of livestock behavior to identify anomalies that may indicate ill health. There is also the use of AI in the form of drones to manage and monitor livestock, thus enabling the collection of real time data on animal health and well being. Some of the companies that are using AI in the livestock industry include Connecterra, Cainthus and HerdDogg who are providing smart solutions for farmers. Another example is IBM’s Watson Decision Platform for Agriculture which is an integrated system that uses AI and advanced analytics to generate insights that farmers can act on.
Benefits
Applying AI in livestock farming has numerous advantages. This is because it supports increased production through the implementation of precision farming and efficient resource management. It improves on animal care through assisting in the detection of diseases at the initial stages and thus preventing the animal from suffering. AI has the potential of also promoting sustainable farming through efficient resource management to reduce waste in livestock farming. In addition, predictive analytics can assist the farmers in their decision making process thereby increasing their profitability and preparedness to risks.
Return on Investment
AI in livestock farming can provide excellent ROI. According to a research done by Intel and the Ponemon Institute, AI has the potential of boosting the return on investment in agriculture to as high as 39% on average by the year 2035. Moreover, the application of precision livestock farming based on artificial intelligence could reduce the costs of livestock production through enhancing the efficiency of resource management. Nonetheless, the ROI is not fixed and may differ based on the particular application, extent of adoption and other considerations. Therefore, although the expenses for implementing AI are substantial, the returns in the future are usually greater than the costs.