
Choose Topic

AI Use Cases
A collection of over 250 uses for artificial intelligence
A continually updated list exploring how different types of AI are used across various industries and AI disciplines,including generative AI use cases, banking AI use cases, AI use cases in healthcare, AI use cases in government, AI use cases in insurance, and more

Sign up
to receive a PDF containing all the use cases and stay updated with the latest AI trends and news (you can always unsubscribe)
Loan decisions
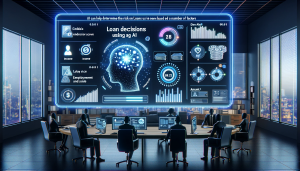
Introduction
The financial services industry has been a major growth engine and is set to undergo a major revolution with the integration of Machine Learning (ML) Artificial Intelligence (AI). The focal area of this transformation is the loan decision-making process. In the past, the process of extending loans has not been an easy one because it involves several factors such as the credit score of the applicant, the income that he or she receives, his or her employment history and many others. But with the integration of ML AI, this process is slowly starting to become more effective and faster. ML AI uses large data sets, computational algorithms, and predictive models to assess the likelihood of a borrower’s default, which makes the loan decision-making process more precise and with lower risks.
Challenges
The application of ML AI in the loan decision making process has some challenges as follows. The first is the question of data privacy and security since AI needs large amounts of personal and financial information. The use of AI comes with concerns of data breaches and inappropriate use of data. Second, there is the problem of bias in AI algorithms. If the data that is fed into the AI is prejudiced in some way then this could result in prejudiced loan decisions. Third, there is the problem of explainability. This is because the decision making process of AI algorithms is often viewed as a black box even by those who are proficient in it. This lack of transparency creates problems with the regulators and the consumers. Finally, there is the problem of how to fit the new technology with the current structures and formations.
AI Solutions
There are, however, a number of challenges that hinder the implementation of AI in the loan decision making process. For example, AI algorithms can be trained to take into account such factors as credit scores, income, and other data to estimate the probability of a customer’s default. Such models can assist the lenders in coming up with better decisions and minimizing on the bad loans. AI can also be applied in the processing of loan applications thereby minimizing the need for manual entry of data and hence faster processing. Also, AI can assist in recognizing hidden correlations and similarities in the data that could not been seen by a human eye, which may be useful in enhancing the decision making of loans. Also, AI solutions can also be used to enhance data security and privacy through the use of encryption and data anonymization.
Benefits
There are a number of advantages of applying ML AI in the loan decision making process. It can help the lenders to make better and faster decisions on loans thus decreasing the chances of bad loans and increasing profitability. It can also enhance the efficiency of the loan application process and create a better relation with the customers. To the borrowers it can grant them a better and equitable loan decision. It also enables them to receive relevant loan proposals that are suitable for their situation. In addition, application of ML AI can enable the development of new and enhanced loan products as well as services since the information derived from the data can be used to create new and improved products and services.
Return on Investment
It is possible to get a good Return on Investment (ROI) when integrating ML AI in the loan decision making process. A research done by Accenture states that AI has the potential of generating $1.2 trillion of value for the financial services industry by 2035. This value is derived from enhanced decision making, risk management, efficiency and experience for the customers. Though, it is crucial to point out that the ROI will depend on several factors such as the quality of data, the complexity of the AI algorithms, and the extent of integration with the current systems and processes.