
Choose Topic

AI Use Cases
A collection of over 250 uses for artificial intelligence
A continually updated list exploring how different types of AI are used across various industries and AI disciplines,including generative AI use cases, banking AI use cases, AI use cases in healthcare, AI use cases in government, AI use cases in insurance, and more

Sign up
to receive a PDF containing all the use cases and stay updated with the latest AI trends and news (you can always unsubscribe)
Material discovery
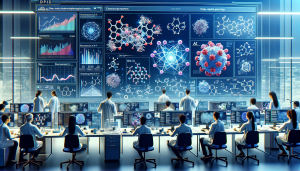
Introduction
The field of materials science which deals with the creation of new materials, has been transformed with the help of artificial intelligence and machine learning. These technologies have the possibility to transform the discovery process and therefore will allow scientists to create better materials for various applications such as energy storage or medical implants. They enable enhanced understanding and forecasting of the properties of materials and hence, help in developing materials with enhanced properties.
Challenges
The creation of new materials has always been a process that involves a lot of time and money. Conventional experimental approaches may take several years to decades to evolve from the discovery phase to the implementation phase. Furthermore, there are a vast number of possible material combinations, therefore the search for new materials is a very complex task. Also, the problem of inaccuracy in predicting properties of new materials is a critical challenge that increases the probability of the approach being more ad hoc than methodical. Also, the absence of a common database for materials science data makes the effective application of the existing information impossible.
AI Solutions
There are several challenges that currently hinder the advancement of the field of materials science, including the cost and time required to discover new materials, the lack of standardization in data collection and reporting, and the issue of data sharing. Here, AI especially ML, presents a viable answer to these problems. Some of the most effective Machine Learning models include Deep Learning and Reinforcement Learning that are capable of learning from large datasets and making good estimations. For instance, the Materials Project, a project that is supported by Berkeley Lab’s supercomputing facilities, applies AI to estimate the properties of all the existing and the new inorganic materials, eliminating the need for a lot of time and resources. Similarly, the company Citrine Informatics apply Artificial Intelligence to guess the properties of new materials and chemicals before they are created. Furthermore, there is an attempt being made by AI-driven platforms such as the Materials Cloud developed by the Swiss Federal Institute of Technology in addressing this problem.
Benefits
There is a large number of benefits that can be derived from the integration of AI into materials science. It speeds up the materials discovery process, which can take years to decades can be reduced to months or even days. Through providing exact predictions of material properties it reduces the chances of the traditional ‘hit-and-miss’ approach, which is cost effective. Also, AI enables the consideration of a larger set of candidates for the materials, which, in turn, enhances the discovery space. Besides, AI facilitates collaboration and data sharing among the researchers and encourages innovation.
Return on Investment
The use of AI in materials discovery can be very profitable. For instance, the creation of new materials for energy storage systems may change the renewable energy industry and create a huge market of 0 billion dollars. In addition, new materials for medical uses may help to enhance the quality of life of patients and decrease the healthcare expenses in the future. Since it is difficult to measure the exact ROI of material discovery due to its wide effect and the fact that it is a long process, the potential returns indicate a high ROI.