
Choose Topic

AI Use Cases
A collection of over 250 uses for artificial intelligence
A continually updated list exploring how different types of AI are used across various industries and AI disciplines,including generative AI use cases, banking AI use cases, AI use cases in healthcare, AI use cases in government, AI use cases in insurance, and more

Sign up
to receive a PDF containing all the use cases and stay updated with the latest AI trends and news (you can always unsubscribe)
Optimise crops based on soil profile
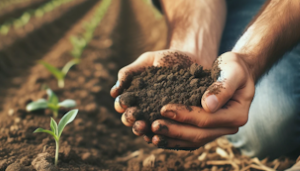
Introduction
Agriculture which is one of the earliest human practices is undergoing a change with the incorporation of artificial intelligence and machine learning. Global population is estimated to reach 9.7 billion by 2050 and food requirement is expected to rise consequently. In response to this, the agriculture sector is adopting artificial intelligence and machine learning to manage the crop production in relation to the soil type. These techniques are applied to many features such as soil characteristics, nutrients, moisture and past production of crops to try to make better predictions. Such system is termed as precision agriculture and is changing the face of farming as we know it.
Challenges
There are however some challenges that the adoption of AI and ML in agriculture is likely to face. This is especially when it comes to the issue of data collection especially in hard to reach areas with poor or no internet connection at all. Another challenge is the absence of a standard in data collection and analysis as is the case in most sectors. This may be due to the fact that farmers may not have the necessary knowledge or skills required to operate complex AI and ML systems. There is also a problem of high initial investment requirements and the inability to determine the ROI. Also, there are ethical and legal concerns such as data privacy and copyright issues which may be problematic.
AI Solutions
There are various AI and ML solutions which are being created to address these issues. Some of the existing examples include AgroScout and Plantix that employ the use of drone technology and artificial intelligence to manage fields, detect pests and diseases as well as offer advice on the appropriate measures to take. IBM’s Watson Decision Platform for Agriculture for instance applies the power of AI to consolidate information and help in decision making within the farming sector. Trace Genomics is a startup which applies ML to analyse soil samples and offer information on the quality of the soil and its effectiveness in crop production. There are other companies for instance Taranis that employ the use of artificial intelligence and machine learning in forecasting weather conditions and how they will affect crop yield.
Benefits
There are many advantages of AI and ML in agriculture. They can enhance efficiency and production through providing accurate forecasts of the yield of crops with the help of soil analysis and other data. They can assist the farmers in deciding when and where to plant, how much fertilizer to apply, and when to harvest. This paper also found that AI and ML can assist in the management of agriculture to minimise the effect of agriculture on the environment through the efficient use of resources and reduction of waste. They can also assist in minimizing the chances of crop failure through poor management such as pests and diseases, or natural factors like adverse weather conditions.
Return on Investment
While the first implementation of AI and ML tools may be expensive, the returns are even more significant. Based on a report by Accenture, AI has the capacity of enhancing productivity in the agriculture sector by forty percent by the year 2035. A study conducted by McKinsey revealed that digital agriculture technologies have the potential of improving crop yields by 20-30% and food waste and supply chain inefficiencies by up to 50%. This has made companies such as John Deere to have high return on investment on their AI and ML technologies.