
Choose Topic

AI Use Cases
A collection of over 250 uses for artificial intelligence
A continually updated list exploring how different types of AI are used across various industries and AI disciplines,including generative AI use cases, banking AI use cases, AI use cases in healthcare, AI use cases in government, AI use cases in insurance, and more

Sign up
to receive a PDF containing all the use cases and stay updated with the latest AI trends and news (you can always unsubscribe)
Patient resource prediction
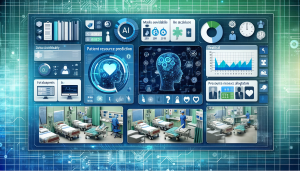
Introduction
The implementation of Machine Learning (ML) and Artificial Intelligence (AI) in the healthcare has been one of the most significant advancements in the sector. These technologies have helped in easing the work of the healthcare practitioners by performing certain tasks, enhancing the diagnostic measures and even predicting the patient’s resource needs accordingly. These two technologies have become an essential part in the management of patient’s resources and they should be in any healthcare setting. The use of these technologies enables the prediction of patient movement, the allocation of resources and even the identification of possible health issues thus enhancing patient outcomes and facility effectiveness.
Challenges
There are, however, some challenges that can hinder the realization of the potential of ML and AI in patient resource prediction. The first challenge is the issue of data quality and availability since to develop accurate predictive models, one needs quality and complete data sets and these are hard to come by particularly due to issues of data privacy and incoherence. The second challenge is the interpretability of ML and AI models. Such models are often opaque, and this makes it hard for the healthcare professionals to comprehend and rely on the recommendations made by the models. The third challenge is how to incorporate such technologies into the current healthcare systems because it may demand alterations in the workflow and procedures. Lastly, there is a possibility of bias in ML and AI algorithms which may result into inequality in health care services and thus aggravate inequalities in health.
AI Solutions
ML and AI have suggested possible solutions to these challenges. For example, federated learning helps multiple institutions to develop a single, accurate model while at the same time, none of the data is shared by the different institutions thus addressing the data privacy issue. Another benefit of AI is that it provides explanations about the decisions made by the AI, which enhances the trust of people in the technology. In addition, the adoption of cloud computing and application programming interfaces (APIs) can help in the implementation of ML and AI into the current healthcare systems. To address the issue of algorithmic bias, there is a growing field of fairness-aware machine learning that aims at delivering equal and fair healthcare services. Predictive analytics is one of the most important developments as it involves the use of historical information to make future estimates. Some of the existing applications include Google’s DeepMind and IBM’s Watson Health which are applying artificial intelligence in assessing risk of patient’s worsening, required resources and likelihood of readmission.
Benefits
The integration of Machine Learning (ML) and Artificial Intelligence (AI) into healthcare has brought significant advancements, particularly in patient resource prediction and flow management. By leveraging vast datasets such as electronic health records (EHRs), ML algorithms can forecast patient admissions, bed requirements, and staffing needs with precision. This enables healthcare providers to optimize resource allocation, avoid overcrowding, and maintain operational efficiency. For example, predictive modeling helps emergency departments and hospitals anticipate capacity issues, improving patient outcomes and overall system performance. These technologies not only streamline operations but also enhance the quality of care, minimizing complications and ensuring timely medical interventions. Moreover, the use of ML and AI facilitates cost savings by reducing unnecessary hospital admissions and lengths of stay. Early disease detection enabled by these technologies allows for preventive measures, alleviating pressure on healthcare systems. AI-powered algorithms can analyze patterns that human analysts might miss, ensuring accurate predictions and better preparedness for future demands. While improving productivity, these innovations also enhance patient experiences by ensuring resources are available when needed. As healthcare systems adopt these transformative tools, they are better positioned to deliver efficient, high-quality, and patient-centered care.
Return on Investment
It is possible to achieve a high ROI when deploying ML and AI in the patient resource prediction process. A study by Accenture reveals that key clinical health AI applications have the potential of realising $150 billion of annual value addition to the U. S. healthcare economy by 2026. Some of the ways through which these savings can be made include; efficient management of resources, minimized admissions, and improved attendance of patients. Nevertheless, the exact ROI may be different based on the particular use case, the effectiveness of the project, and how prepared the organization is for such an change.