
Choose Topic

AI Use Cases
A collection of over 250 uses for artificial intelligence
A continually updated list exploring how different types of AI are used across various industries and AI disciplines,including generative AI use cases, banking AI use cases, AI use cases in healthcare, AI use cases in government, AI use cases in insurance, and more

Sign up
to receive a PDF containing all the use cases and stay updated with the latest AI trends and news (you can always unsubscribe)
Patient risk prediction
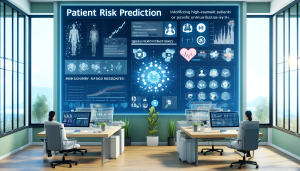
Introduction
Machine Learning (ML) and Artificial Intelligence (AI) have been two of the most used technologies in the recent years and have brought about great changes. Another area that has greatly been affected by these changes include the healthcare sector. In particular, these technologies have demonstrated a high level of possibility in the area of patient risk assessment. Patient risk prediction is a method that is used to determine the patients who are likely to have certain diseases or conditions. The goal is to enable the identification of risks in order to intervene early and provide treatment, so as to enhance the quality of patient care. With the use of ML and AI, it is now possible to make predictions of risks more accurately, generate individualized care plans, and therefore enhance the results of patients.
Challenges
There are however some issues that have to be solved in order to realize the potential of ML and AI in patient risk prediction. First, the healthcare data is usually stored in isolated systems and therefore difficult to integrate and analyse. Second, legal requirements such as the HIPAA in the U. S or the GDPR in Europe restrict the use of patient information. Third, there is a shortage of well-structured data in the healthcare sector, which hinders the application of ML and AI. Fourth, the healthcare personnel are generally not receptive to new technologies and this may hinder the adaptation of the AI tools. Lastly, ML and AI models are data intensive and sometimes it is difficult to collect large quantity of quality data.
AI Solutions
There are several challenges that AI has to face in order to implement the use of EHRs and these include; To tackle these challenges, AI has offered the following solutions. For fragmented data, AI can employ Natural Language Processing to extract meaningful information from an unstructured data. For privacy issues, Federated learning can be applied where an algorithm is trained across a number of individual devices or servers that hold local data samples, without sharing the data. For standardization, AI can transform various types of data into a single format. To address lack of trust from the healthcare professionals, AI companies are developing easy to use interfaces and providing training. In case of a big data problem, AI can use synthetic data or transfer learning to train its models with fewer data. Also, there are other companies such as IBM Watson Health and Google’s DeepMind Health developing AI tools for patient risk assessment.
Benefits
There are a lot of benefits of using ML and AI for patient risk assessment and they are as follows. They can also result in better predictions thus enabling the identification and treatment to be made at the earliest. They can also be used in patient care to help in determining how individual patients will likely react to certain treatments. They can also reduce costs by identifying the patients who are most likely to develop complications and require intensive care. Furthermore, they can enhance the management of the patients by forecasting the movement of patients in the hospital. Lastly, they can allow for a proactive care since one can be able to identify the upcoming health issues.
Return on Investment
The cost of investment of AI in patient risk prediction can be lucrative. Based on a research done by Accenture, application of AI in healthcare can potentially reduce costs by up to $150 billion for the US healthcare economy by 2026. McKinsey has also predicted that big data and ML in pharma and medicine has the potential to create a value of $100 billion on an annual basis. In addition, a research published in the Journal of Medical Internet Research revealed that an AI system developed to estimate the patient’s life expectancy did better than the conventional approaches, thus highlighting the possibilities of reduced costs and enhanced patient outcomes.