
Choose Topic

AI Use Cases
A collection of over 250 uses for artificial intelligence
A continually updated list exploring how different types of AI are used across various industries and AI disciplines,including generative AI use cases, banking AI use cases, AI use cases in healthcare, AI use cases in government, AI use cases in insurance, and more

Sign up
to receive a PDF containing all the use cases and stay updated with the latest AI trends and news (you can always unsubscribe)
Pest identification
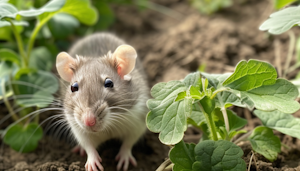
Introduction
The agriculture industry which is one of the strongest industries in the world has been struggling with the problem of effective pest control. The new and improved use of Machine Learning (ML) and Artificial Intelligence (AI) has brought about a new dimension in the detection and identification of pests thus changing the face of pest control. With the help of AI and ML it is now possible to detect and recognize the pests quickly and correctly, which leads to the application of the proper preventive measures before it is too late and the damage is done. This technology uses sophisticated algorithms to identify patterns and learn from large sets of data that may include pictures of the pests, their development and the havoc they wreck.
Challenges
There are however some challenges that can hinder the effectiveness of the use of AI and ML in pest identification. Some of them are; the absence of adequate amount of quality data that is labeled to train the ML models; the reliability of internet connection in the farming sector especially in the remote areas; the challenge that comes with the process of developing and installing AI solutions including the time and the money; and farmers’ perceptions and acceptance of the new technologies. In addition, the variability of the pests, their different growth phases, and the numerous conditions where they occur also pose a challenge to the development of the general AI solutions.
AI Solutions
In order to address these problems, a number of AI solutions have been designed. For example, IBM has come up with ‘AgroPad’ that employs AI and ML to determine the presence of pests in soil samples while ‘FarmVisionAI’ incorporates the use of deep learning to manage crops and identify pests. Also, there is ‘PestSmart Diagnostics’, a CABI’s initiative that applies AI to recognize crop pests and diseases in East Africa. Other examples include several AI-based mobile applications such as ‘Plantix’ and ‘SmartFarm’ which assist farmers to quickly determine the pests they have. These solutions incorporate sophisticated image processing algorithms to process pictures of crops and recognize any discordant parts which may indicate the presence of harmful organisms. They also incorporate predictive analytics in predicting the occurrence of pest incidence so that the farmers may be prepared for it.
Benefits
There is a wide range of applications of AI and ML in pest management. The utilization of AI and ML in pest identification facilitates a fast and precise process of identifying the pests, which in turn minimises the loss of crops. Through predication of pest epidemics it enables farmers to prevent the outbreak of pests and with that reduce the use of damaging pesticides. The technology can also assist in assessing the impact of the pest control measures that are being undertaken in the farms by giving real time feedbacks and allowing changes to be made where necessary. Furthermore, it can also enhance the efficiency of farming and make farming sustainable and also contribute towards food security and environmental conservation.
Return on Investment
It is estimated that the use of AI and ML in pest identification can have high returns on investment. Although, the main disadvantage of implementing these technologies is that it may require a lot of money to set up initially, the advantages that can be gained in terms of improved crop yields, reduced pesticide usage and improved operational efficiency may be able to offset these costs. According to a research done by Accenture, AI has the potential of increasing agricultural productivity by 25%, while a report by PwC revealed that AI applications in agriculture can generate up to $5 trillion of the global GDP by 2030. However, the ROI would vary based on many factors such as the size of the farm, the crop to be planted, the intensity of pest infestation and the efficiency of the AI/ML solution.