
Choose Topic

AI Use Cases
A collection of over 250 uses for artificial intelligence
A continually updated list exploring how different types of AI are used across various industries and AI disciplines,including generative AI use cases, banking AI use cases, AI use cases in healthcare, AI use cases in government, AI use cases in insurance, and more

Sign up
to receive a PDF containing all the use cases and stay updated with the latest AI trends and news (you can always unsubscribe)
Predicting market demand
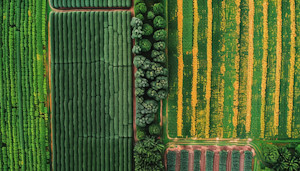
Introduction
The use of ML and AI technologies in the agricultural sector is a great example of how integration of technology can bring positive change in the food production sector. There is a great potential for the implementation of ML and AI in agriculture as it has the possibility of revolutionalizing the face of agriculture through improved production, efficient resource management and increased returns on investment. Specifically, these technologies are increasingly being applied in the ability to forecast the market demand for particular type of crop or livestock product which is a crucial factor for farmers, agribusinesses, and policymakers. Thus, the accurate market demand forecasting can help to minimize the losses from food waste, to optimize the resources usage, and to obtain important information for management decision-making.
Challenges
There are, however, a number of barriers that the agricultural industry faces in the implementation of these technologies. First, a major challenge is the scarcity of quality, reliable and timely data which is vital for the training and validation of ML models. Second, the nature of agricultural markets is quite volatile and cannot be easily predicted as they depend on various factors including weather conditions, diseases, pests, and even the overall state of the economy. Third, there is a shortage of human resources who have knowledge and skills in agriculture on one hand and artificial intelligence and machine learning on the other hand, this affects the creation and adoption of these technologies. Finally, there is a requirement for huge investment in the technology infrastructure which can be a challenge for the small farmers and agribusinesses.
AI Solutions
These challenges are addressed by AI and ML with the help of technological advancements. Predictive analytics which is based on the ML algorithms can analyse large volumes of data to recognise trends that could be hard for a human to identify. AI can also work with multiple data sets including satellite images, weather data and even prices in the market to enhance the accuracy of demand estimations. The use of deep learning algorithms makes it possible to capture complex correlations that are otherwise difficult to establish particularly because agricultural markets are rather volatile. Also, AI can do the work of analyzing data and this eliminates the need for a person to be involved and this means that costs are also reduced. Some of the companies include IBM with Watson Decision Platform for Agriculture and aWhere which provide intelligent solutions for agricultural predictions.
Benefits
There are several advantages of using AI and ML in the prediction of agricultural market demand. It can also help to enhance efficiency and profitability by aiding farmers and agribusinesses to make better decisions based on the data presented. It can also help in proper utilization of resources as in predicting the demand for particular crops hence minimizing on waste and increasing efficiency. It can also increase the stability of the agricultural sector since it will provide an early warning on possible changes in the market condition which will enable the stakeholders to change their strategies. Also, it can enhance food security by bridging the gap between supply and demand of agricultural products and thus minimizing the occurrence of famines or stockpiling of certain agricultural products.
Return on Investment
The ROI of AI and ML in estimating market demand for agriculture can be very valuable. A report by McKinsey has stated that AI has the potential of enhancing agricultural output by 60%. Also, a research by AlphaBeta revealed that digital agriculture which includes AI and ML could increase the value of crops globally to $500 billion. Nevertheless, the ROI can be very high but with different rates for each application, data quality, and amount of investment on technology, infrastructure and training. Despite the initial expenses, the sustainable advantages such as efficiency, profitability, and resilience make the use of AI and ML in the agricultural sector worthwhile.