
Choose Topic

AI Use Cases
A collection of over 250 uses for artificial intelligence
A continually updated list exploring how different types of AI are used across various industries and AI disciplines,including generative AI use cases, banking AI use cases, AI use cases in healthcare, AI use cases in government, AI use cases in insurance, and more

Sign up
to receive a PDF containing all the use cases and stay updated with the latest AI trends and news (you can always unsubscribe)
Predictive ETAs for shipments
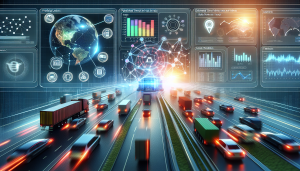
Introduction
Predictive Estimated Time of Arrival (ETA) is one of the most crucial aspects in the field of logistics as accurate forecasting can greatly improve efficiency, reduce costs and increase customer satisfaction. It can be defined as the use of advanced mathematical models and computer programs to estimate the time that a shipment is expected to arrive at its destination. Recently, the use of ML and AI has been recognized as game changers that can revolutionize the accurate forecasting of predictive ETAs. These technologies are capable of working with vast amounts of information, getting smarter with every new entry, and producing reliable projections which can help the companies in the logistics business to improve their performance and offer more effective solutions to their clients.
Challenges
There are several barriers that the logistics industry encounters in providing accurate Predictive ETAs. Some of these are weather conditions, traffic jam, and mechanical failures of the vehicles. Such challenges cannot be easily solved by manual methods of prediction since they are rather complex and stochastic in nature. Also, the conventional analytics tools do not have the ability to cope with the large volume of data produced by current logistics operations. Other challenges include, the challenge of data integration where there are no seamless data flows between the various systems and the costs of purchasing, implementing and maintaining AI solutions.
AI Solutions
These challenges can be solved with the help of AI and ML. There are many types of machine learning algorithms that are capable of analyzing large data sets, shipment history data, real time traffic information and weather prediction to make right estimations. This is because AI can also consolidate data from various systems and eliminate data silos to give a bigger picture of the logistics processes. In addition, the AI solutions are proactive; they get better with the more data they analyse. For instance, DHL has come up with a machine learning model that helps in estimating the delay in air freight transportation to enhance the effectiveness of operations. Likewise, IBM has also come up with an AI-based solution namely IBM Sterling Supply Chain Insights that applies machine learning to identify and mitigate risks in the supply chain.
Benefits
There is vast potential for the application of AI and ML in enhancing the accuracy of predictive ETAs. Some of the key benefits include enhanced accuracy that leads to improved performance and reduced costs. This is because by knowing the delays that are likely to occur, logistics companies can be in a position to take preventive measures such as changing the route for shipment or adjusting the schedule. This can help in improving the customers’ satisfaction through timely deliveries. It also has the capability of identifying patterns and trends in the data that would otherwise be difficult to recognize. Additionally, through automation, AI releases the employees to work on other tasks thus increasing efficiency.
Return on Investment
In this case, the return on investment (ROI) of AI and ML in predictive ETAs can be very profitable. A study by McKinsey has revealed that AI can cut forecasting errors by almost half and reduce lost sales by as much as 65% through effective management of out of stock situations. In addition, the automation of basic tasks can lead to huge cost savings. Nevertheless, the ROI can be different in its magnitude and sign depending on the particular application, data quality, and the complexity of the AI system. Hence, it is important for companies to assess their requirements and choices so as to make wise investments in AI and ML.