
Choose Topic

AI Use Cases
A collection of over 250 uses for artificial intelligence
A continually updated list exploring how different types of AI are used across various industries and AI disciplines,including generative AI use cases, banking AI use cases, AI use cases in healthcare, AI use cases in government, AI use cases in insurance, and more

Sign up
to receive a PDF containing all the use cases and stay updated with the latest AI trends and news (you can always unsubscribe)
Predictive maintenance for fleet management
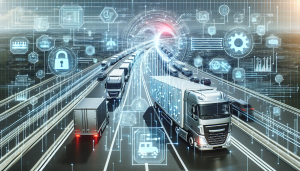
Introduction
ML and AI have become an important part of many industries, logistics being one of them. Of all the applications, fleet management has greatly benefited from the use of ML and AI especially through predictive maintenance. Predictive maintenance leverages data from IoT sensors, past maintenance records, and even environmental conditions to predict when equipment is likely to fail, thus enabling organizations to shift from breakdown maintenance to preventive maintenance. This approach enables the enhancement of the reliability and availability of equipment, effective cost management and minimizing downtime.
Challenges
There are however certain challenges that are still hindering the implementation of predictive maintenance. Some of these are the challenge in accumulating and assimilating large data, the challenge in consolidating different data sets, the challenge in finding skilled professionals to manage and interpret the data, and the challenge of investing heavily to integrate predictive maintenance systems. Also, there is a problem of ensuring the safety and confidentiality of the data that is being gathered and the problem of making people in charge understand and agree on the advantages and return on investment of predictive maintenance. Since these are companies that manage large fleets, FedEx and UPS have had to deal with these problems as they adopt predictive maintenance.
AI Solutions
AI and ML have become indispensable in the area of predictive maintenance for the fleet management. AI-based solutions are capable of processing large data sets, pattern recognition and making reliable predictions of equipment failure. For example, UPS has implemented AI to forecast mechanical problems in its vehicles and thus perform the necessary maintenance before a failure occurs. Likewise, FedEx employs ML to analyse data gathered from sensors fitted in its vehicles with a view of predicting and preventing equipment failure. They can also assist in decision making since the solutions provide an analysis of the ROI of maintenance activities as well as the right time to carry out maintenance. Furthermore, AI can also assist in the area of data security and privacy by putting in place strong security measures and adhering to data guidelines.
Benefits
The following are the advantages of using predictive maintenance in fleet management. This paper thus; helps in avoiding unexpected down time through equipment failure prediction hence increasing efficiency and reducing costs. Predictive maintenance also enhances the reliability and the overall life of equipment therefore reducing costs. Furthermore, through maintaining equipment, predictive maintenance also helps in preventing equipment failures, an issue that is of utmost concern in the logistics industry. Amazon and DHL are some of the companies that have adopted predictive maintenance and they have seen great improvements in productivity, cost and safety.
Return on Investment
Predictive maintenance in fleet management can provide a high return on investment (ROI). A study by PwC revealed that predictive maintenance can cut down on maintenance expenses by 30%, enhance the lifespan of equipment by 20% and decrease downtime by 50%. This has been acknowledged by companies such as UPS and FedEx that have seen cost reductions and enhancement in operational effectiveness due to predictive maintenance. However, the ROI is not fixed and is influenced by several factors such as the size of the fleet, the type of equipment in use, and the level of adoption of predictive maintenance.