
Choose Topic

AI Use Cases
A collection of over 250 uses for artificial intelligence
A continually updated list exploring how different types of AI are used across various industries and AI disciplines,including generative AI use cases, banking AI use cases, AI use cases in healthcare, AI use cases in government, AI use cases in insurance, and more

Sign up
to receive a PDF containing all the use cases and stay updated with the latest AI trends and news (you can always unsubscribe)
Predictive maintenance for network infrastructure
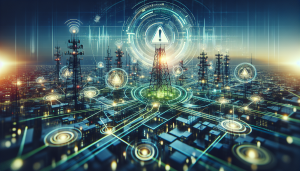
Introduction
In the modern world of telecommunications, organizations are constantly in the pursuit of enhancing the performance of the network as well as improving the reliability of the network while at the same time looking for ways of cutting costs. Another important area of focus is the predictive maintenance of the network infrastructure. Classically, the network maintenance has been based on a reactive approach where issues are addressed when they occur. But this approach results in prolonged downtime, high repair costs, and low customer satisfaction. Predictive maintenance, which is based on the use of Machine Learning (ML) and Artificial Intelligence (AI) is a more proactive method. This strategy involves the use of sophisticated algorithms in determining possible failures and inefficiencies before they happen therefore enabling appropriate measures to be taken. These two concepts have changed the face of predictive maintenance by offering accurate real time analysis of network to enable operators to coordinate maintenance activities, increase equipment’s life and improve service delivery.
Challenges
There are, however, some challenges that can hinder the realization of the potential that is offered by the implementation of ML and AI in predictive maintenance. Some of these challenges include the challenge that is associated with the integration of AI systems with other structures, the challenge that comes with the collection of large amounts of high-quality data that are required to train the AI models, and the challenge that is associated with the understanding of AI predictions. In addition, there are issues of data privacy and security, and also the cost of acquiring AI technology is quite high. Especially, most of the telecom companies suffer from the shortage of in-house experts in AI, which makes it difficult to create, deploy, and control efficient AI systems. Also, there is the problem of creating trust in AI systems by the employees and consumers as they may have little or no faith in the technology or worries about it taking their jobs.
AI Solutions
There are however several challenges that hinder the effectiveness of predictive maintenance in the telecom industry. Such challenges include; These range from complex data analytics tools that are capable of identifying trends and anomalies in the network performance data to sophisticated machine learning algorithms that are capable of predicting failures of equipment as well as network outages. For instance, IBM and Microsoft have come up with AI platforms that can be easily incorporated with other systems, are capable of processing large data sets in real time and generating useful information for preventive maintenance. AI can also assist in performing regular maintenance tasks thus reducing the workload of the employees to focus on more important tasks. In addition, AI solutions can be integrated with other technologies including IoT and cloud computing to improve their results. For instance, smart IoT sensors can gather information from network devices in real-time, and such data can be analyzed using cloud computing platforms and AI.
Benefits
There are a number of reasons why ML and AI are beneficial in predictive maintenance specifically for the telecom industry. Some of the advantages include minimization of down time since potential problems can be detected and solved before they become network failures. This can in turn enhance the quality of service and the customers’ satisfaction. This is because AI can also be used to increase the useful life of network equipment through effective maintenance scheduling thus minimizing replacement needs. In addition, AI can also help in increasing the efficiency of the operations by performing routine tasks which in turn enable the staff to work on important issues. In addition, AI enables better and timely decision making since AI systems are capable of processing large data sets faster and with more precision as compared to humans. Last but not the least, by enhancing maintenance efficiency and service quality, AI can provide telecom companies the edge they need in the competition.
Return on Investment
While it is true that the deployment of AI technology may require a significant initial capital, the returns can be quite immense. As reported by McKinsey, predictive maintenance can cut down on maintenance expenses by 20-40%, increase equipment’s useful life by 20%, and decrease downtime by up to 50%. Also, an Accenture research revealed that AI has the capacity of increasing profitability within the telecommunications sector by 38% by 2035. The ROI will, of course, vary depending on such factors as the extent of the deployment, the quality of data that goes into developing the AI models, and the success of the AI solution. Nonetheless, there is a strong argument to be made for AI for predictive maintenance based on the possibility of huge cost reductions and enhanced service delivery.