
Choose Topic

AI Use Cases
A collection of over 250 uses for artificial intelligence
A continually updated list exploring how different types of AI are used across various industries and AI disciplines,including generative AI use cases, banking AI use cases, AI use cases in healthcare, AI use cases in government, AI use cases in insurance, and more

Sign up
to receive a PDF containing all the use cases and stay updated with the latest AI trends and news (you can always unsubscribe)
Predictive supply chain risk management
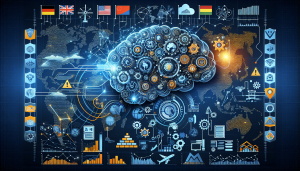
Introduction
Logistics is an important part of the global trade since it is through logistics that the movement of goods and services is made across the world. Still, it is not immune to certain challenges. From the impacts of the global events to the dynamics of the supply chain processes, managing risks in the logistics industry is not an easy task. The logistics industry is rapidly evolving due to technological advancements such as machine learning (ML), generative artificial intelligence (AI), and predictive analytics. These technologies offer the tools to forecast and reduce supply chain threats and thus enhance the performance of the logistics processes.
Challenges
There are a number of challenges that the logistics industry has to deal with in order to effectively mitigate supply chain risks. Some of these are operational challenges that include inventory management, demand forecasting and capacity planning among others. On a macro level, geopolitical risks, natural disasters and health risks can have a severe impact on the supply chains. Also, the growing complexity of supply chains where there are many interlinked parts and participants located in various countries increases the risk. This paper examines how the traditional approaches to risk management are inadequate for handling these diverse and integrated risks in a effective and timely manner.
AI Solutions
AI and ML have revolutionalized the management of supply chain risks in the logistics industry. The predictive analytics which is enhanced by the AI and ML can use large data sets to search for trends and potentials to happen in future thus enabling preventive measures to be taken. For instance, it is possible to apply AI to estimate the demand and improve the supply chain management to avoid stockouts or excess inventory. Some of the benefits include; ML can be used to analyze historical shipment information with a view of forecasting possible delays and altering the schedule. Risk assessment tools that incorporate the use of Artificial Intelligence are capable of assessing the risk profile of suppliers and make recommendations on how to manage the risk. Also, the AI platforms are capable of tracking the dynamic data from various channels and identify the supply chain issues and set the response process in motion. Some of the big companies such as IBM and Maersk are already applying AI and blockchain in developing more efficient and effective supply chains.
Benefits
There are a number of ways in which the application of AI and ML can be beneficial in the area of supply chain risk management. The use of predictive analytics can therefore be used to enhance demand forecasting thus minimizing the chances of stockouts as well as over stocking which may be costly. Supply chain management can be improved through the use of artificial intelligence, this means that identification and management of disruptions will be enhanced. This can minimize downtime and thus loss of sales. It also has the potential of enhancing supplier risk management and ensure that there is a stable and reliable supply chain. In particular, the adoption of AI and ML can enhance the effectiveness and robustness of the supply chain management processes and increase customer satisfaction and financial performance.
Return on Investment
There are significant returns on investment (ROI) when using Artificial Intelligence (AI) and Machine Learning (ML) in supply chain risk management. For example, McKinsey’s research shows that AI-enabled supply chain management can cut forecasting errors by almost half, which translates to reduction in lost sales due to product unavailability by 65%. The same study also revealed that AI can cut supply chain administrative costs by as much as 40%. In addition, the enhanced visibility and predictability that comes with AI can be used to minimize the occurrence of costly supply chain disruptions and enhance customer satisfaction, both of which are positive for revenue. Hence, even though initial investment on AI and ML solutions may be costly in the short run, the future returns can be extremely beneficial for many companies.