
Choose Topic

AI Use Cases
A collection of over 250 uses for artificial intelligence
A continually updated list exploring how different types of AI are used across various industries and AI disciplines,including generative AI use cases, banking AI use cases, AI use cases in healthcare, AI use cases in government, AI use cases in insurance, and more

Sign up
to receive a PDF containing all the use cases and stay updated with the latest AI trends and news (you can always unsubscribe)
Prognosis creation from diagnosis
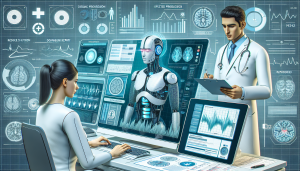
Introduction
In the current world of fast developing healthcare sector, creation of prognosis from diagnosis has become one of the most important aspects. Prognosis creation process has been greatly enhanced by the use of AI technologies such as Risk Assessment Graphics (RAG). RAG AI integrates machine learning algorithms to assess and interpret the vast amount of information that is collected in the field of medicine, helping the practitioners to provide better and more precise patient outcome predictions. It has been used in a variety of areas such as the assessment of disease progression and in the formation of individualized treatment plans.
Challenges
There are however several challenges that can hinder the realization of RAG AI in prognosis creation. First, the issue of data completeness and quality is an important factor as the medical data collected may be incomplete, inaccurate or unstructured especially in the case of high-dimensional data. Second, the issue of data integration is hampered by the absence of standardization in medical data recording and storage systems. Third, since medical data is considered to be very sensitive, there are issues of privacy and security that need to be addressed in order to protect the patients’ information. Fourth, the healthcare professionals may not easily embrace the new technology since they may have little or no knowledge on it or they may think that it will pose a threat of taking their jobs. Finally, there are regulatory issues that may be a problem since the use of AI in healthcare is a highly compliant area.
AI Solutions
There are however several AI solutions that have been put in place to solve some of these problems. For example, deep learning models can work with high dimensional data and thus provide better results. Some examples include IBM’s Watson Health which applies AI in analyzing and organizing complex and disparate medical information. To tackle the issue of privacy, there is the differentially private model and other strict security measures are being taken. In order to break through the resistance being offered by the healthcare professionals, there is currently efforts being made to create awareness on the advantages of AI as well as how it can complement the work of the professionals instead of substituting them. The following are some of the steps that are being taken to deal with regulatory issues; there is always a process of engaging the regulatory authorities to define the rules of the use of AI in healthcare.
Benefits
There are numerous advantages of applying RAG AI in the creation of prognosis. It increases the precision and the efficiency of the prognosis as well as the timeliness of the interventions. It provides specific treatment plans for an individual by forecasting the dynamics of his or her disease. Through the analysis of large data sets, it is possible to identify similarities and connections which may enable the discovery of new aspects of disease aetiology. Lastly, it can minimize the cost of healthcare through enhancing the efficiency of treatment as well as avoiding the unnecessary interventions.
Return on Investment
The integration of RAG AI into healthcare offers significant benefits, including reducing administrative workloads, enhancing patient access, and improving health outcomes. Studies by Accenture and McKinsey highlight the potential for AI to save billions annually in healthcare costs by improving efficiency and care quality. AI can reduce administrative tasks by over 50%, allowing healthcare professionals to focus more on patient care while improving scheduling efficiency. Furthermore, AI enhances health outcomes through better treatment plans, progress monitoring, and faster drug discovery, with Deloitte estimating a 25% improvement in drug development processes. Despite these advantages, challenges like data privacy, organizational resistance, and skill gaps pose barriers to widespread adoption. Patients express concerns about the security of their data, as highlighted by the Kaiser Family Foundation, while staff resistance and legacy systems complicate AI integration. Addressing these issues requires a clear adoption strategy, robust data governance, and targeted training programs, as suggested by Boston Consulting Group and Gartner. With continued advancements, RAG AI is poised to revolutionize healthcare, with the global AI healthcare market expected to grow to $6.18 billion by 2025, paving the way for transformative impacts in efficiency, research, and patient care.