
Choose Topic

AI Use Cases
A collection of over 250 uses for artificial intelligence
A continually updated list exploring how different types of AI are used across various industries and AI disciplines,including generative AI use cases, banking AI use cases, AI use cases in healthcare, AI use cases in government, AI use cases in insurance, and more

Sign up
to receive a PDF containing all the use cases and stay updated with the latest AI trends and news (you can always unsubscribe)
Quality control
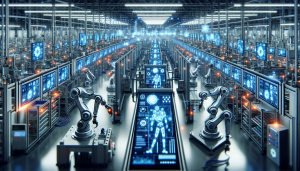
Introduction
Quality control is a critical aspect of the manufacturing industry. It involves maintaining a high standard of products by identifying and correcting defects before they reach the end consumer. Traditionally, quality control has been a labor-intensive and time-consuming process, requiring significant human intervention. However, in recent years, machine learning (ML) and artificial intelligence (AI) technologies have been increasingly applied to automate and enhance the quality control process. This integration of ML and AI in quality control has transformed the manufacturing industry, enabling companies to achieve higher standards of quality, efficiency, and productivity.
Challenges
The manufacturing industry faces several challenges in quality control. Firstly, the traditional manual inspection process is prone to human error and inconsistency. Secondly, it is difficult to maintain the same level of quality across different batches of products due to variability in manufacturing processes. Thirdly, detecting defects in complex products can be challenging due to their intricate designs and the need for precise measurements. Finally, the increasing demand for personalized products requires manufacturers to constantly adapt and update their quality control processes, which can be costly and time-consuming.
AI Solutions
AI and ML technologies offer innovative solutions to these challenges. For instance, AI-powered visual inspection systems can automatically detect and classify defects in products, reducing human error and increasing consistency. ML algorithms can analyze data from manufacturing processes to predict and prevent potential defects, enabling proactive quality control. AI can also be used to create digital twins of products, allowing for virtual testing and validation before actual production. Moreover, AI and ML can facilitate adaptive manufacturing by continuously learning and improving from data, enabling manufacturers to efficiently produce personalized products. Companies like IBM and Microsoft have developed comprehensive AI solutions for quality control in manufacturing.
Benefits
The integration of ML and AI in quality control brings numerous benefits to the manufacturing industry. Firstly, it enhances the accuracy and consistency of quality control by reducing human error. Secondly, it increases productivity and efficiency by automating inspection processes. Thirdly, it enables proactive quality control through predictive analytics, reducing the occurrence of defects and recalls. Fourthly, it facilitates adaptive manufacturing, allowing manufacturers to efficiently produce personalized products. Finally, it improves customer satisfaction by ensuring high-quality products.
Return on Investment
The return on investment (ROI) of integrating ML and AI in quality control can be significant. For example, a study by Capgemini found that AI can increase operational efficiency by up to 20%. Moreover, a report by Accenture estimated that AI could add $3.7 trillion to the manufacturing sector by 2035. The ROI can also be seen in the reduction of costs associated with defects and recalls, the increase in productivity, and the improvement in customer satisfaction.