
Choose Topic

AI Use Cases
A collection of over 250 uses for artificial intelligence
A continually updated list exploring how different types of AI are used across various industries and AI disciplines,including generative AI use cases, banking AI use cases, AI use cases in healthcare, AI use cases in government, AI use cases in insurance, and more

Sign up
to receive a PDF containing all the use cases and stay updated with the latest AI trends and news (you can always unsubscribe)
Readmission risk prediction
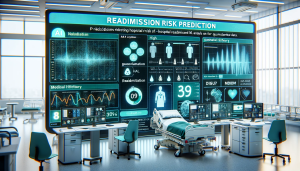
Introduction
The use of ML and AI has revolutionized many industries and fields, and healthcare is one of them as well. Especially in the field of readmission risk assessment, AI has displayed tremendous potential. Hospital readmissions not only lead to the expenditure of more resources but also may suggest that there is something wrong with the care that was provided. Through the use of estimations of a patient’s readmission possibility, healthcare professionals can act accordingly to improve the patient’s condition and minimize the spending. Due to the ability to work with large amounts of data, as well as to comprehend the relationships between various parameters and make predictions, ML and AI algorithms are perfect for the task.
Challenges
There are however some barriers that can hinder the use of AI in readmission risk prediction in healthcare. The first and probably the most important issue is the problem of data availability and data quality. To make accurate predictions one needs a large amount of high quality data which is usually hard to come by especially due to the issues of patient data privacy and disjointed healthcare systems. Second, there is a problem of handling various types of medical data that may be both organized as in the case of patient age or unorganized as in the case of clinical documents. Third, there is the issue of interpretability of AI models; it is crucial for clinicians to know how and why the predictions are made in order to make proper choices. Last but not the least, there are other factors which can act as barriers to the integration of AI including funding, skill limitations, and compliance issues.
AI Solutions
To address these issues, different AI solutions have been suggested. Some of the methods that can deal with the complexity and variance of medical information include ensemble learning models, deep learning models, and natural language processing models. For instance, Google’s DeepMind Health is creating AI algorithms that can interpret medical photographs and the progression of diseases. IBM’s Watson Health uses AI to capture and analyze both, structured and unstructured data with a focus on patient’s outcome. In order to make the AI predictions more understandable to humans there is a developing field called Explainable AI (XAI). Also, the use of cloud-based AI can help minimize the costs and the need for initial investment and hardware, which may be advantageous for small healthcare facilities.
Benefits
There are numerous advantages of applying AI in readmission risk assessment. It allows for identification of the high risk patients who need intervention before they are discharged from the hospital, thus reducing the risk of admissions. It can also assist in the management of resources especially in the identification of the high risk patients who need special attention. In addition, AI can help to reveal the characteristics that have not been noticeable at the first sight, thus providing a better understanding of readmission processes. For instance, the UCSF’s AI system, which was described above, found new predictors of heart failure readmission. It can also perform monotonous tasks thus freeing the health care professionals to attend to the patients.
Return on Investment
There is a high ROI when it comes to applying AI in readmission risk assessment. A study by Accenture states that AI applications in healthcare may create a financial impact of up to $150 billion by 2026 in the United States only. More specifically, AI assisted early intervention can therefore be seen to offset $20 billion annually. Also, the monetary gains that can be made from minimizing readmissions are quite impressive. For instance, a case study from Mount Sinai Hospital shows that applying AI in patient readmission prediction helped the hospital to decrease the number of readmissions by 56% within a 10 month period which translates to cost savings. In addition, the intangible gain from enhanced patient outcome and experience can also be quite high.