
Choose Topic

AI Use Cases
A collection of over 250 uses for artificial intelligence
A continually updated list exploring how different types of AI are used across various industries and AI disciplines,including generative AI use cases, banking AI use cases, AI use cases in healthcare, AI use cases in government, AI use cases in insurance, and more

Sign up
to receive a PDF containing all the use cases and stay updated with the latest AI trends and news (you can always unsubscribe)
Recommending relevant clinical trials or research studies based on patient profiles
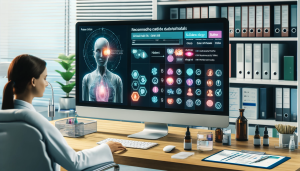
Introduction
In the last decade, the healthcare industry has evolved quickly because of the adoption of technologies in the delivery of healthcare services. Of these, there are two major categories that have gained prominence in transforming the healthcare management and discovery processes: Machine Learning (ML) and Artificial Intelligence (AI). There are specific domains where there is a growing use of AI and ML and one of them is in suggesting suitable clinical trials or research studies to patients based on their characteristics. Clinical trials are the building blocks of every medical development. It is a way of determining the efficacy of new drugs, treatment, and interventions. Still, it is very difficult to identify the right candidates for these trials. This is where AI and ML step in, assisting in the process of linking patient’s characteristics with appropriate clinical trials effectively and timely.
Challenges
There are many barriers that the healthcare industry encounters when it comes to identifying the right clinical trials for patients according to their characteristics. The process is often a labor intensive, time consuming and a error prone process. This involves the process of sorting through large data sets to find the right patients for the right trial based on their medical history, diseases they have, and even their genetics. In addition, there is a possibility of bias when patients are selected manually and some groups of people may be excluded. Another issue is that clinical trials and patients are constantly changing and thus the information needs to be updated on a regular basis. There is also the issue of data privacy since health information is considered to be sensitive.
AI Solutions
There are several challenges that affect the efficiency of matching patients to suitable clinical trials in the precision medicine arena. Here, AI and ML offer viable answers. Some of the most important benefits include; Machine learning algorithms are capable of analyzing large data sets within the shortest time and with a high level of precision that can be hardly achieved by a human being. In this view, AI can greatly enhance the patient trial matching process through data analysis and minimising the need for human input. One of the most advanced forms of ML is deep learning that enables the ML algorithms to handle structured data like genetic data and make the patient trial matching process more precise. It also has the potential of eliminating biases that may arise in the patient selection process through providing a more holistic and non-biased analysis of the patient’s data. This can be achieved by applying secure AI frameworks that help in the protection of patient information such as through data privacy. For example, IBM’s Watson Health is an AI-powered tool that identifies trials that a particular patient may be eligible for based on the patient’s data.
Benefits
There are a number of ways in which the use of AI and ML for suggesting suitable clinical trials to patients on the basis of their profiles can be advantageous. The following are some of the benefits that may be achieved from the use of these technologies in patient-trial matching: They can also help to save time and money in the process of patient-trial matching since they can work faster and with a higher level of precision. They can also improve the inclusion of different people in the clinical trials, which makes the results of the trials to be more reliable. Personalized recommendations is another benefit of AI, to enhance the care of patients and improve their results. It also has the potential of enhancing the process of identifying new therapies and treatments by improving the conduct of clinical trials. Also, AI can help to improve the security of data and privacy of patients’ information as shown below.
Return on Investment
It can be advantageous to employ ROI when implementing AI and ML in recommending clinical trials to patients according to their demographics. Hospitals and research institutions can benefit from the reduction of time and resources, which results to cost efficiency. The improvement in the efficiency and effectiveness of the clinical trials will enable faster time to market for new therapies and treatments thus increasing revenue. It has also been found that better patient outcomes can also lead to lowering of healthcare costs in the future. Furthermore, the application of AI can improve the image and the competitive edge of the healthcare organizations in terms of attracting funds and collaborations.