
Choose Topic

AI Use Cases
A collection of over 250 uses for artificial intelligence
A continually updated list exploring how different types of AI are used across various industries and AI disciplines,including generative AI use cases, banking AI use cases, AI use cases in healthcare, AI use cases in government, AI use cases in insurance, and more

Sign up
to receive a PDF containing all the use cases and stay updated with the latest AI trends and news (you can always unsubscribe)
Soil health monitoring
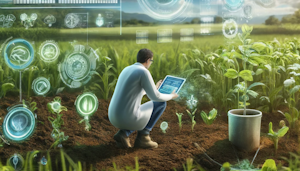
Introduction
Soil is one of the most essential natural resources that exist on the planet and it covers almost one third of the earth’s surface and it is estimated that about 70% of the earth’s fresh water is groundwater that is stored in the soil. Therefore, the health of the soil is a very important factor which has an effect on food production, quality of water and even plays a role in the regulation of the climate. Hence, it is important to undertake soil health monitoring as a key factor in maintaining the health of the environment and the sustainability of the agricultural sector. Machine Learning (ML) and Artificial Intelligence (AI) have emerged as powerful tools in the recent times and have started to be used in soil health monitoring as well to analyse and assess the soil conditions in new ways. The potential that these technologies provide to farmers, researchers and policy makers in the understanding and management of soil health as well as the practice of sustainable agriculture and food security in the future can hardly be overemphasized.
Challenges
There are, however, a number of challenges that are associated with soil health monitoring. The conventional soil testing techniques are cumbersome, slow and do not always give a detailed account of the state of the soil at any given time. Also, such methods do not capture the depth and scope of information regarding soil health and the factors that influence it. Soil differences within various fields, shifts in climate conditions, and the requirement for prompt decisions pose a difficulty as well. Another obstacle is how to integrate and interpret large quantities of data collected from various sources and make them useful for decision making. Last but not least, the application of modern technologies in the rural and underdeveloped areas can be limited by the absence of the necessary infrastructure, expertise, and equipment.
AI Solutions
These challenges are being addressed by AI and ML with the help of intelligent solutions. There is the use of AI enhanced soil sensors that can collect large amounts of data on the soil conditions at specific points in time, for instance nutrients, moisture, temperature, pH and many others. The machine learning algorithms then can use this information to recognise trends, forecast the conditions and suggest measures for land management. For example, IBM has come up with AgroPad, an eight millimeter thick hardware that incorporates AI to help in the analysis of soil samples and give real time results on the health of the soil. Likewise, new companies like Trace Genomics apply ML to analyze soil DNA and offer insights on disease threats and fertility of the soil. In addition, it is possible to connect AI with other types of information, including weather predictions, remote sensing images, and data received from IoT devices to create a better understanding of the state of the soil. Other application include the use of machine learning models in forecasting the effect which different management practices or even variation in environment would have on soil health to assist in decision making and planning.
Benefits
There is a wide range of applications of AI in soil health monitoring and management which has the following advantages. This makes the process of soil testing faster and accurate thus reducing the time and effort needed for the exercise. Some of the benefits of using AI include the provision of real-time data as well as predictive analytics which can assist farmers to make proper and timely decisions to improve on crop yields and quality. It also enables the identification of soil health risks at the right time to facilitate the prevention or management of soil diseases and degradation hence maintaining soil fertility and biodiversity. Also, the capability of AI in collecting and combining large data sets can support the understanding of soil health and the development of sustainable agricultural policies. As a result, the application of AI in the management of soil health and status through remote sensing in the future can help in enhancing food security, environmental conservation, and climate change adaptation.
Return on Investment
It is possible to see how the application of AI in soil health monitoring can generate a high ROI. Although, the implementation of the AI technologies at the initial level may be costly, the returns in the future may be substantial. According to the research, precision farming that involves the use of AI in soil monitoring can boost crop production by 20%. Other costs that can be cut include reduction in fertilizer and pesticide usage, decreased labor expenses, and avoidance of soil erosion. In addition, the worth of healthier soils and sustainability is difficult to calculate in monetary terms but is incredibly valuable. In the context of the global goals for sustainable development, the application of artificial intelligence in the management of soil health is a powerful way of supporting the objectives of ending hunger, ensuring access to clean water, and conserving the terrestrial ecosystems.