
Choose Topic

AI Use Cases
A collection of over 250 uses for artificial intelligence
A continually updated list exploring how different types of AI are used across various industries and AI disciplines,including generative AI use cases, banking AI use cases, AI use cases in healthcare, AI use cases in government, AI use cases in insurance, and more

Sign up
to receive a PDF containing all the use cases and stay updated with the latest AI trends and news (you can always unsubscribe)
Species identification
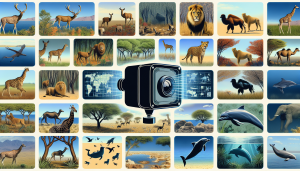
Introduction
The use of Machine Learning (ML), Computer Vision (CV) and Artificial Intelligence (AI) in the identification of species has been changing the face of the wildlife industry. It creates new ways of carrying out research, assessing animal numbers, and designing protection strategies. These technologies make it possible to automate the process of species identification thus allowing for faster data acquisition and analysis. It also means that researchers and conservationists can be able to track and comprehend wildlife population, their activities, and their range. However, there are some issues that affect the integration of these technologies in the wildlife industry.
Challenges
There are various challenges that one is likely to encounter in the integration of ML, CV, and AI in species identification. This implies that there is a need for a lot of data and quality data as well to train these models. In addition, it is also a challenge to collect enough data especially in the case of wildlife because some species are difficult to capture or the environment may be unfavorable. There are also issues of privacy and ethical issues since the use of AI tools in wildlife areas may disrupt natural behaviour or environments. In addition, there is a problem of vast number of species which makes it complicated to develop algorithms that can recognize all of them. Finally, there is also a lack of capacity in the field of wildlife conservation for the use and application of these technologies.
AI Solutions
These are the challenges that AI solutions are intended to address. For example, social media platforms such as iNaturalist and eBird use machine learning and computer vision to help users identify species from their photos and sounds. Microsoft has also come up with AI for Earth which provides cloud and AI solutions to organizations that are addressing issues in the environment such as species identification. One of the popular services is from the giant tech company Google, namely AI platform AutoML Vision that has been applied in the development of models that are used to recognize wildlife in the images captured by trail cameras. Wildbook is a software framework which implements AI to recognise animals based on their ‘biometric’ characteristics like colour of their stripes and monitor them across time. These solutions use highly effective algorithms and large data sets to distinguish and categorise various species to improve the studies and protection activities.
Benefits
There are numerous advantages of employing ML, CV and AI in species identification. They help in faster and efficient identification of species thus eliminating the need of many people and minimizing the chances of mistakes. They also allow for the tracking of specific animals over time, which helps to understand more about the animal’s life history and behavior. In addition, these technologies are capable of working through large amounts of information in a short time and thus enhance research and conservation. Through applying the concept of species identification through automation, the researchers are able to spend more time on analyzing and interpreting the results which in turn will help in developing better conservation measures.
Return on Investment
There is a high return on investment (ROI) of applying ML, CV and AI in species identification. Although there is an initial expense that has to be incurred in order to develop the necessary infrastructure and to train the models, the returns are sustainable. For example, the time and resources that would have been used for traditional identification can be reduced, thus leading to cost efficiency. In addition, the enhanced efficiency of data collection and analysis as well as the increased accuracy of the data collected can help in developing better conservation measures. This could therefore have a financial payoff, since better health of ecosystems can lead to more tourism and other related industries. Also, the value of biodiversity cannot be overemphasized even though it is difficult to put a price tag on it.