
Choose Topic

AI Use Cases
A collection of over 250 uses for artificial intelligence
A continually updated list exploring how different types of AI are used across various industries and AI disciplines,including generative AI use cases, banking AI use cases, AI use cases in healthcare, AI use cases in government, AI use cases in insurance, and more

Sign up
to receive a PDF containing all the use cases and stay updated with the latest AI trends and news (you can always unsubscribe)
Spot suspicious or anomalous transactions
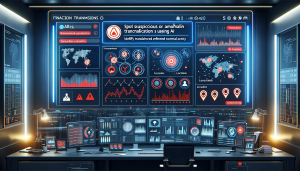
Introduction
In the ever-evolving financial services industry, combating fraud is a critical priority as the volume and complexity of transactions increase. Traditional detection methods are no longer sufficient, prompting financial institutions to adopt Machine Learning (ML) and Artificial Intelligence (AI). These technologies analyze vast datasets to identify patterns and anomalies that signal potential fraud, offering real-time detection and adaptive capabilities to address new types of fraudulent activities. Data analytics complements this effort by uncovering hidden trends, enabling earlier detection of suspicious behavior, and minimizing the impact of financial crimes. While ML and AI provide significant advantages in fraud prevention, they also present challenges such as data privacy concerns, algorithmic bias, and the need for explainable AI decisions. Regulators are working to ensure ethical and transparent use of these technologies through guidelines and accountability measures. Case studies, such as those from Barclays and J.P. Morgan, demonstrate the transformative impact of AI and ML in financial crime prevention. As these technologies advance, their integration into regulatory frameworks and institutional processes will enhance their effectiveness, paving the way for a more secure and transparent financial ecosystem.
Challenges
There are several difficulties which the financial services industry faces in identifying suspicious transactions. These are; improved strategies by the financial criminals, enhanced transaction throughput, complex nature of the transactions, the requirement to make the detection and prevention in real time, and the problem of differentiation between the legitimate and illicit activities. Another major challenge is the high false positive rate of the conventional methods that are used, which results in increased investigation time and customer unhappiness. Also, the issue of meeting the ever changing compliance standards is also another challenge.
AI Solutions
AI and ML are changing the face of financial crime prevention in the financial sector. These technologies employ a number of algorithms to assess large sets of transaction information in real time and uncover any discrepancies which may be suspicious of fraud. It can also adapt to new types of transactions and update itself based on the previous transactions that it has seen. The most common AI methods which are applied to this question are supervised learning, unsupervised learning, deep learning, and reinforcement learning. For instance, HSBC has collaborated with Ayasdi for the use of AI in the automated process of anti-money laundering compliance. Also, Mastercard has implemented the use of AI in the analysis of transactions with an aim of identifying any suspicious patterns.
Benefits
There are numerous advantages of utilizing artificial intelligence and machine learning in identifying suspicious transactions. The speed and efficiency of fraud detection can be improved greatly with the help of these technologies hence reducing costs. They can also lower the rate of false positives, which makes customers happy and reduces the amount of time spent on investigations. In addition, it can assist the financial institutions in meeting the standard regulatory obligations and also offer important information that can be applied to enhance the risk appraisal and decision-making procedures. For instance, Danske Bank has made a revelation that it has cut down the number of false positives by 60% while at the same time increasing the ability to detect genuine fraud by 50% after adopting an AI-based fraud detection system.
Return on Investment
There is a high return on investment (ROI) when implementing AI and ML in fraud detection. A study by McKinsey & Company revealed that AI can minimize fraud rate of transactions to as low as 40%, thus reducing costs. Also, since AI helps in minimizing the number of false positives, it also improves the customer experience and loyalty, which is a benefit to financial institutions. Also, by applying AI for fraud detection, financial institutions can prevent or reduce the incidence of fines and reputation loss, which can also be counted as an investment return.