
Choose Topic

AI Use Cases
A collection of over 250 uses for artificial intelligence
A continually updated list exploring how different types of AI are used across various industries and AI disciplines,including generative AI use cases, banking AI use cases, AI use cases in healthcare, AI use cases in government, AI use cases in insurance, and more

Sign up
to receive a PDF containing all the use cases and stay updated with the latest AI trends and news (you can always unsubscribe)
Tornado prediction
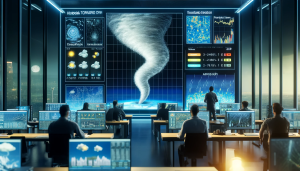
Introduction
In the field of environmental sciences, it has always been difficult if not impossible to forecast as well as follow the movements of tornadoes. Tornadoes are an example of an extreme weather event that can cause destruction and fatalities and is a type of natural disaster that can cause loss of property. The traditional approach to tornado forecasting requires a good deal of knowledge of meteorological parameters which are often subject to a certain level of error and variability. However, there is increasing use of ML and AI in enhancing the efficiency and the precision of the tornado prediction and forecasting. Using the techniques of AI and ML, it is possible for scientists and meteorologists to work through large data sets, identify trends, and provide forecasts that are more reliable.
Challenges
The problem of tornado forecasting is the fact that tornadoes are very hard to predict due to the dynamics of weather conditions. Tornado formation and development is a function of a number of factors that include temperature, humidity, wind speed and direction among others. A second problem is that accurate predictions require a large number of data. Weather stations produce millions of data every day and it takes a lot of time and effort to analyze such data using conventional approaches. There are also problems concerning the data accuracy and reliability since meteorological data may be inaccurate and erratic. This is because there is no enough tornado data for modeling which is a big challenge as the models need a lot of data to train on.
AI Solutions
There are various challenges that these challenges. AI and ML offer innovative solutions to these challenges. There are types of machine learning and one of them is the deep learning that can handle large amount of data and identify complicated patterns, which is great for identifying tornadoes. The researchers at the University of Oklahoma and the National Severe Storms Laboratory have come up with a machine learning model known as ‘TORUS’ which is capable of forecasting tornadoes one to two hours in advance. The model operates on radar information and meteorological factors to make its forecasts. In a similar manner, IBM's ‘GRAF’ application integrates AI to offer high-resolution temporal enhanced forecasts of weather conditions including the possibility of tornadoes.
Benefits
There are many ways in which AI and ML can be useful in enhancing the prediction of tornadoes. They can help to increase the precision and the efficiency of the predictions thus enabling the issuance of earlier warnings that can help in preventing loss of lives. Another benefit of AI is that it can work through data much faster and with greater precision as compared to the conventional techniques thus leaving more time for the meteorologists to work on other tasks. Also, AI improves as it goes, this means that the more data it gets the better the predictions it will make. For instance, IBM’s GRAF system has been estimated to have provided 200% improvement in the accuracy of the traditional forecasting systems.
Return on Investment
It can be difficult to determine the ROIs of AI in tornado prediction, but it is almost certain that the cost savings are immense. Through providing earlier and more accurate warnings, AI can minimize the effects of tornadoes thereby cutting down on the costs of reconstruction and recovery. In addition, AI can also result in efficiency gains where the data analysis and prediction process is streamlined and less labour intensive. Furthermore, the enhanced accuracy of the predictions can also contribute to the enhancement of trust and confidence in weather forecasts, which may in turn enhance the utilization of the forecast services and products.