
Choose Topic

AI Use Cases
A collection of over 250 uses for artificial intelligence
A continually updated list exploring how different types of AI are used across various industries and AI disciplines,including generative AI use cases, banking AI use cases, AI use cases in healthcare, AI use cases in government, AI use cases in insurance, and more

Sign up
to receive a PDF containing all the use cases and stay updated with the latest AI trends and news (you can always unsubscribe)
Trend prediction
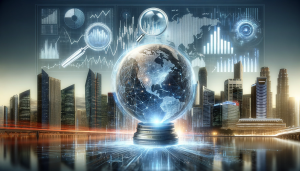
Introduction
Trend prediction is a crucial aspect of decision-making in the financial services industry, enabling organizations to effectively manage risks, invest wisely, and make informed market decisions. Recent advancements in Machine Learning (ML) and Artificial Intelligence (AI) have revolutionized this process by offering powerful tools to analyze vast datasets, uncover trends, and detect anomalies that are beyond human capability. These technologies enhance the accuracy and efficiency of trend prediction, providing actionable insights that support strategic planning and operational excellence in a competitive market. Despite their potential, integrating ML and AI into financial services presents challenges, including data privacy concerns, over-reliance on technology, data quality issues, and the need for skilled professionals. Addressing these challenges requires robust data cleaning and validation, adherence to ethical guidelines, investment in infrastructure, and continuous learning to stay ahead of rapid technological changes. By balancing human expertise with AI-driven insights and ensuring transparency in AI decisions, organizations can harness the full potential of these technologies, driving innovation and maintaining a competitive edge in the dynamic financial sector.
Challenges
There are however some challenges that the application of ML and AI in trend prediction in the financial services industry is likely to encounter. The first challenge is the issue of data; the quality and the amount of data. This means that if these algorithms are not fed with enough quality and properly arranged data, they will not be in a position to make good forecasts. Third, while making use of these models, there is a possibility of over optimization whereby the model becomes very large and complex thus reducing its ability to make good predictions. Last but not the least, there are issues of accountability and fairness of these models, as well as legal and moral questions that come with them.
AI Solutions
There are several challenges that can be addressed by AI and ML. They are capable of dealing with big data and unstructured data using techniques such as deep learning and reinforcement learning. Some of the algorithms that are effective in handling high dimensionality and reducing overfitting include XGBoost and LightGBM. This is where natural language processing comes in to incorporate other factors that cannot be quantified through numbers such as news reports and social media trends into the models. Lastly, there are other methods like SHAP and LIME which can enhance the accountability and interpretability of these models.
Benefits
There are a lot of advantages of incorporating AI and ML in the detection of trends in the financial industry. It can enhance the efficiency and the precision of the predictions that are made, which in turn can result to enhanced decision making and enhanced financial results. It can also assist in the risk management aspect in that it will be able to pinpoint any possible economic upturn or downturn in the market. In addition, they can assist in the decision-making process and help in the reduction of the need for human input and possible mistakes. Last but not the least, they can also assist in the enhancement of the compliance and regulatory reporting as clear and easily understood models and predictions.
Return on Investment
It is possible to obtain a good return on investment (ROI) when applying AI and ML to trend forecasting in the financial sector. Although, the implementation of such technologies may require a significant amount of capital, the enhanced financial outcomes and lower risks can sometimes generate high returns. A research done by Accenture state that, financial institutions that have implemented AI have gained 34% boost in revenue. Also, the costs reduction due to automation and less human factors can also be counted as the ROI.