
Choose Topic

AI Use Cases
A collection of over 250 uses for artificial intelligence
A continually updated list exploring how different types of AI are used across various industries and AI disciplines,including generative AI use cases, banking AI use cases, AI use cases in healthcare, AI use cases in government, AI use cases in insurance, and more

Sign up
to receive a PDF containing all the use cases and stay updated with the latest AI trends and news (you can always unsubscribe)
Visual defect detection
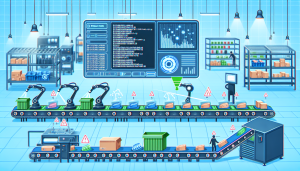
Introduction
AI is a game-chiner in various industries and manufacturing is one of them. Of which, ML, which is the category of AI, has been widely applied in the visual defect detection in manufacturing. This involves the use of sophisticated mathematical computations and AI models to enable a computer to be able to pick out and categorize defects in products during the production process. There is therefore the benefit of effectively and efficiently identifying defects as this enhances product quality and at the same time reduces production costs and time. While the conventional approaches were mostly labor-based and involved the use of human inspectors, ML AI has been identified as a better option that can perform the task with more precision and efficiency, including identifying flaws that may be undetectable to the human eye.
Challenges
There are, however, some challenges that can hinder the effective implementation of ML AI in visual defect detection in the manufacturing industry even though it has a high potential. This is an important factor because to train the ML models, one requires a large amount of high quality data. Furthermore, the current manufacturing processes are complex in nature, and this results in various kinds of defects which makes it difficult for the ML models to identify all the defects with ease. Other obstacles are as follows: Integration of ML models with the current manufacturing processes, expensive costs, and shortage of experts who will operate and maintain such systems. In addition, there are challenges that arise from data privacy and security since most company information that is sensitive is used to develop ML models.
AI Solutions
Despite these challenges, there has been the development of several AI solutions that can be used for visual defect detection in manufacturing industries. For example, Convolutional Neural Networks (CNN), a type of deep learning model, has been found to be effective in image and video analysis and, therefore, suitable for visual defect detection. Big pharma companies such as IBM has also come up with solutions such as IBM Maximo Visual Inspection that uses AI to detect and categorize defects with a high level of accuracy. Similarly, Google’s AutoML Vision is also an AI solution which enables manufacturers to train their own ML models for image classification task that the manufacturers want the images to be classified into. Another such solution is Microsoft’s Project Bonsai which is an AI platform for developing autonomous systems which can also be used by manufacturers for developing AI models for defect detection.
Benefits
The process of choosing the right cameras and lighting for the manufacturing line is very vital in the implementation of the ML AI. When choosing the cameras there are factors that need to be put into consideration such as the resolution that is needed to identify defects and the capacity to take pictures at high speeds if the line is fast. Lighting is also as crucial as the other factors as it makes sure that the images are properly lit and without shadows hence may not give a correct positive or negative result. Appropriate lighting can also help to improve the contrast of defects thus making it easier to identify them.
Return on Investment
The return on investment (ROI) for implementing ML AI in visual defect detection can be substantial. While the initial cost of implementing such systems can be high, the long-term benefits often outweigh these costs. According to a report by McKinsey, the use of AI in quality control can lead to a 50% reduction in defect rates, translating into significant cost savings. Additionally, the time saved by automating defect detection can be used to enhance productivity and innovation. Furthermore, the improved product quality resulting from accurate defect detection can lead to increased customer satisfaction and loyalty, thus boosting sales and profits. Therefore, while the exact ROI may vary depending on the specific circumstances of each manufacturer, the potential for significant financial gains is clear.