
Choose Topic

AI Use Cases
A collection of over 250 uses for artificial intelligence
A continually updated list exploring how different types of AI are used across various industries and AI disciplines,including generative AI use cases, banking AI use cases, AI use cases in healthcare, AI use cases in government, AI use cases in insurance, and more

Sign up
to receive a PDF containing all the use cases and stay updated with the latest AI trends and news (you can always unsubscribe)
Weather pattern decisions
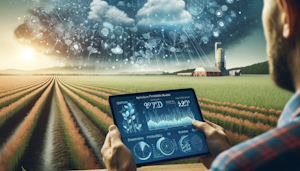
Introduction
Agriculture has been an essential part of the society for thousands of years as it fulfills the needs of people and allows societies to develop further. In the recent past, agriculture has been faced with several challenges such as fluctuating weather conditions as a result of climate change, and pressure from population increase leading to high food demand. To address these challenges, the industry has relied on technology and specifically on machine learning (ML) and artificial intelligence (AI). These technologies have the capacity of transforming the agricultural sector and help farmers to be well prepared on the weather patterns and other environmental conditions. The following is a comprehensive analysis of the modern applications of ML and AI in weather patterns in the agriculture sector.
Challenges
There are many issues that are faced by the agriculture industry and these can be aggravated by weather conditions. Some of them are natural disasters such as drought, floods and storms that can ruin crops; variation in climate patterns that affect the normal crop yield and crop pests; and the challenge of efficient resource management. Since the greater part of farming is still done the traditional way, such challenges result in reduced harvest, high costs, and famine. Also, there is a limited access to accurate and timely information regarding the weather conditions and the state of the crops which makes farmers unable to act appropriately and address the problems that occur.
AI Solutions
There are several challenges that can be solved by adopting ML and AI. For instance, by employing predictive models, AI can be utilized to assess past weather information and create reliable predictions. These models can also provide information about the identification of trends that can be used to predict the future weather conditions. ML techniques can be applied to images to track the state of crops and potentially difficulty that can occur in advance. Some of the applications include optimizing on the amount of water, fertilizer and other resources that are required for each crop type given the current and future weather conditions through the use of AI. Some of the big companies such as IBM have come up with Watson Decision Platform for Agriculture to enhance the development of these AI solutions.
Benefits
There are a number of ways in which ML and AI can be useful in the decision-making process of weather patterns in agriculture. Some of the advantages are; improved crop production through the assistance of ai in scheduling planting and harvesting times according to the weather conditions; reduced costs through the optimization of resources; and increased food security through the ability of ai in forecasting and minimizing effects of adverse weather conditions. Also, AI can offer the farmers real-time information and analysis and hence they can make better decisions and address issues promptly.
Return on Investment
It can be argued that the use of ML and AI in weather pattern decisions in agriculture can be profitable in terms of the ROI. For instance,research done by McKinsey and Company revealed that the adoption of digital agriculture technologies such as AI could improve crop production by up to 60% and minimize food loss through wastage by up to 20%. Also, research done by PwC revealed that AI has the potential of adding $5.7 trillion to the global economy by 2030 and agriculture being one of the sectors that can benefit most from it. Some of the real-life examples of successful ROI are companies such as Understory that offers hyperlocal weather information to farmers and has been estimated to enhance crop production by between 10 and 20%.