
Choose Topic

AI Use Cases
A collection of over 250 uses for artificial intelligence
A continually updated list exploring how different types of AI are used across various industries and AI disciplines,including generative AI use cases, banking AI use cases, AI use cases in healthcare, AI use cases in government, AI use cases in insurance, and more

Sign up
to receive a PDF containing all the use cases and stay updated with the latest AI trends and news (you can always unsubscribe)
Wildlife health monitoring
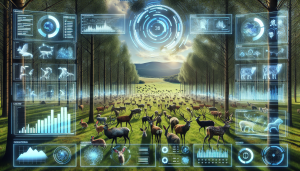
Introduction
Given the current state of the world and the ongoing threats to our environment, wildlife monitoring has become an important branch of science. This is a branch that has mainly depended on such fundamentals as camera traps, GPS collars, and people to watch for the data. The last decade, however, has seen the integration of Machine Learning (ML) and Artificial Intelligence (AI) technologies in the process and now they are revolutionizing the approach. They provide a way of doing this efficiently, accurately and faster through the automation and enhancement of the process. With the use of artificial intelligence and machine learning in this area, it has made it easier for conservationist and researchers to protect endangered species, and also maintain the balance of the ecosystem.
Challenges
However, there are some challenges that one is bound to encounter while implementing AI and ML in the monitoring of wildlife health. The first challenge is the issue of data; the problem of how to capture, store and manage the large quantities of high quality data that are required for these technologies to work. This data is normally collected in hard to access areas and more often collected in the course of challenging circumstances which makes it hard to collect, store and analyze. Also, the vast amount of data that is captured from camera traps, drones and tracking devices can be very time consuming and difficult to organise and interpret by hand. In addition, there is a shortage of the required computational power and skilled personnel in the conservation sector to enable the proper application and utilization of these technologies. Finally, there are ethical issues associated with the application of AI and ML in wildlife monitoring including concerns over animals’ privacy and effects of the monitoring equipment on animal behavior.
AI Solutions
Despite these challenges, several AI and ML solutions have been designed and implemented to improve the current state of wildlife health monitoring. For instance, the ‘AI for Earth’ project by Microsoft applies AI and big data solutions for solving problems related to earth. Some of the applications include the use of ML to recognize species from the camera trap images, control of animal populations, and even detection of disease and stress in animals. Another example is Google’s ‘Wildlife Insights’ which uses AI to break down millions of camera trap images and give information about the wildlife species and their actions. Also, ‘Conservation Metrics’ employs the use of machine learning models to analyze audio information to determine species of birds and bats as well as their population size. Also, ‘Wildbook’, an open-source software platform, applies AI to distinguish between specific animals and to record their behavior and their contacts in space and time.
Benefits
There are many advantages of using AI and ML in the field of wildlife health monitoring. This is because it has the potential of enhancing the efficiency and speed of data processing thus enabling real time tracking and identification of potential threats. It also enhances the precision of species recognition and estimation of the population size thus minimizing the chances of mistakes that could be made by humans. Also, it provides the capability to observe the wildlife on a broader and more complex scale as has never been done before, thus giving better picture of various habitats. Finally, AI and ML can essentially perform a large part of the data collection and analysis, which in turn can ease the burden on the conservationists and enable them to concentrate more on the practical aspect and policy making.
Return on Investment
It can be argued that the use of AI and ML in the monitoring of wildlife health is a good investment decision as far as the ROI is concerned. This is because while a lot of money may be required to set up these technologies in the beginning, the benefits in terms of time, resources and even manpower can be immense in the future. In addition, the capabilities of these technologies to identify problems at the initial stage and with high level of precision can avert expensive and possibly irreparable losses of life of wildlife and deterioration of habitats. For instance, the AI technology in the ‘AI for Earth’ has been estimated to cut the time it takes to process an image from 30 minutes to 30 seconds, which is a huge savings in terms of time and effort.