
Choose Topic

AI Use Cases
A collection of over 250 uses for artificial intelligence
A continually updated list exploring how different types of AI are used across various industries and AI disciplines,including generative AI use cases, banking AI use cases, AI use cases in healthcare, AI use cases in government, AI use cases in insurance, and more

Sign up
to receive a PDF containing all the use cases and stay updated with the latest AI trends and news (you can always unsubscribe)
Wound healing image analysis
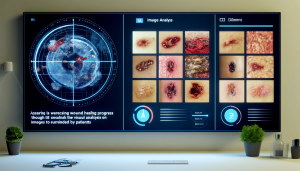
Introduction
There are many components which are involved in the wound healing process in a sequential manner, including cellular structures. Wounds are not constant; they vary in their appearance, the cause of their development and may be accompanied by comorbidities, which makes their management by health care providers difficult. To this end, there has been an increasing interest in applying Computer Vision (CV) and Artificial Intelligence (AI) in the analysis of wound healing images. CV AI has the capacity to change the current methods of assessing and therefore, managing wounds as it is accurate in measuring the size and shape of wounds, the time it will take for a wound to heal and identifying complications earlier. This technology is currently being used in the healthcare sector and has shown great potential.
Challenges
There are several barriers in wound healing image analysis. First, wound images can be obtained under different lighting conditions, using different cameras and at different angles, which makes the images vary considerably. Second, the edges of wounds are not always easily discernible, thus making it difficult to determine the actual size and depth of the wound. Third, comorbidities such as diabetes or vascular diseases may affect the healing process and thus influence the analysis. Fourth, since there are no well-defined protocols for wound imaging and assessment, the evaluations are not only inconsistent but also subjective at times. Last, there are issues of privacy and security when it comes to managing patient images and information.
AI Solutions
These challenges are solved by AI especially CV. For example, Swift Medical’s AI solution uses a smartphone camera to take wound photos and analyze them. It defines the wound area, computes the size of the wound and monitors the evolution of the wound. Tissue Analytics is another company which applies machine learning models to categorize the wounds and to estimate the time for healing. MolecuLight i:X is a fluorescence imaging device which incorporates the use of Artificial Intelligence to help in the identification of bacteria in wounds which are key to the healing process. Project InnerEye is an AI tool for image analysis developed by Microsoft and is capable of being extended for the purpose of wound assessment. In addition, it is shown that AI can assist in the process of wound assessment to achieve greater accuracy and less inter and intra rater discrepancy. By incorporating patient information such as the medical history and lifestyle factors, AI can also offer a better assessment of the wound healing process.
Benefits
There are many advantages of AI in wound healing image analysis. It enhances the reliability of the measurements made for wounds thus enhancing the accuracy of the treatment plans. It also has the potential of identifying complications at an early stage thus decreasing the admission and surgery rates. It is also capable of outlining the wound healing expectations and help in patient counseling as well as prediction of the wound healing time. It allows for the remote management of wounds which is especially useful in the community setting and in times of outbreak. The following are some of the ways through which AI can help in research and education to improve on the understanding of wounds and their management.
Return on Investment
The ROI of using AI in wound healing image analysis can be substantial. By improving wound assessment and management, AI can help reduce healthcare costs associated with prolonged hospital stays, surgeries, and complications. A study conducted by the University of Manchester estimated that wound care costs the UK healthcare system up to £5.3 billion annually. By enhancing wound healing rates and outcomes, AI can contribute to significant savings. Moreover, AI can increase productivity by saving clinicians' time spent on wound assessment, allowing them to focus on patient care. ROI can also be seen in terms of improved patient satisfaction and outcomes.